In Episode 15 of Sidecar Sync, Amith and Mallory , delve into Neuralink's brain chip implantation, generative AI's privacy risks, and New York City's Local Law 144 on AI in hiring. Discussions focus on the potential and challenges of these developments in artificial intelligence, with insights on their implications for associations and nonprofits.
Let us know what you think about the podcast. Drop your questions or comments in the Sidecar community: https://community.sidecarglobal.com/c/sidecar-sync/
Join the AI Learning Hub for Associations: https://sidecarglobal.com/bootcamp
Download Ascend: Unlocking the Power of AI for Associations: https://sidecarglobal.com/AI
Join the CEO AI Mastermind Group: https://sidecarglobal.com/association-ceo-mastermind-2024/
Thanks to this episode’s sponsors!
AI Learning Hub for Associations: https://sidecarglobal.com/bootcamp
Tools/Experiments mentioned:
Team-Chat GPT: https://team-gpt.com/
Microsoft Co-pilot: https://www.microsoft.com/en-us/microsoft-copilot/
Betty Bot: https://bettybot.ai/
Topics/Resources Mentioned:
Neuralink brain chip impant: https://www.cnn.com/2024/01/30/business/elon-musk-brain-implant-neuralink-intl-hnk/index.html#:~:text=Elon%20Musk%27s%20controversial%20startup%20Neuralink,was%20recovering%20well%2C%20he%20added.
Data Privacy and Generative AI: https://www.infosecurity-magazine.com/news/banning-generative-ai-privacy-risks/
NYC Local Law 144: https://www.natlawreview.com/article/nyc-s-local-law-144-and-final-regulations-regulation-ai-driven-hiring-tools-united
EU AI Act: https://artificialintelligenceact.eu/the-act/
Social:
Follow Sidecar on LinkedIn: https://www.linkedin.com/company/sidecar-global
Amith Nagarajan: https://www.linkedin.com/in/amithnagarajan/
Mallory Mejias: https://www.linkedin.com/in/mallorymejias/
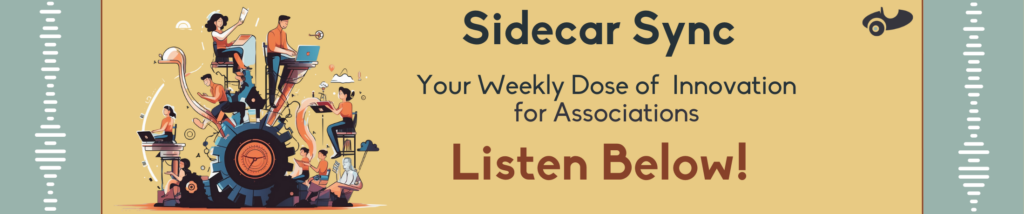
This transcript was generated by artificial intelligence. It may contain errors or inaccuracies.
Amith Nagarajan: [00:00:00] So if this chip has access to your brain and it therefore potentially could access your memories, if that's something the chip could do at some point, that becomes a concern, which you really want a chip to be able to access your long term memory bank. Welcome to Sidecar Sync, your weekly dose of innovation. If you're looking for the latest news, insights, and developments in the association world, especially those driven by artificial intelligence, you're in the right place. We cut through the noise to bring you the most relevant updates, with a keen focus on how AI and other emerging technologies are shaping the future.
No fluff, just facts and informed discussions. I'm Amith Nagarajan, chairman of Blue Cypress, and I'm your host.
Greetings everybody. And welcome back to the sidecar sync. We are on episode 15 today and we have a bunch of really exciting, interesting topics to go over with you. I'm really excited to be back with my [00:01:00] cohost Mallory, and we're going to jump right in. Now a word from our sponsor.
Mallory Mejias: Today's sponsor is the Sidecar AI Learning Hub. If you are looking to dive deeper on your AI education in 2024 and beyond, I encourage you to check out Sidecar's AI Learning Hub. With the bootcamp, you'll get access to flexible, on demand lessons, and not only that. Lessons that we regularly update. So you can be sure that you are keeping up with the latest in artificial intelligence.
You'll also get access to weekly live office hours with our AI experts, and you get access to a community of fellow AI enthusiasts in the association and greater nonprofit space. You can get the bootcamp for 399 a year on an annual subscription, and you can also get access for your whole team for one flat rate.
If you want more information on Sidecar's AI Learning Hub, go to sidecarglobal. com slash bootcamp
Amith, how are you doing today?
Amith Nagarajan: I'm doing great. It's exciting to have good weather in New Orleans and I'm looking forward to this conversation. How [00:02:00] about you?
Mallory Mejias: Absolutely. I'm doing pretty well. And today is more of the type of episode that we have done in the past. We are focusing on three kind of top news items from last week. And this week I'm excited for it. The topics we are talking about today are Neuralink. Which is Elon Musk's new company that just implanted a chip into a human brain for the first time.
We'll be talking about generative AI and privacy risks in honor of Data Week, which was last week. And then we'll be talking about New York City's AI hiring law and how successful or not successful that might have been.
Amith Nagarajan: Sounds exciting. A lot of interesting things to cover there. And uh, you know, the whole idea of AI bridging into the real world is going to be a fun thing to dig into, particularly in that first topic.
Mallory Mejias: Well, let's dive right in. So, let's talk about Neuralink. We are diving into the recent and remarkable milestone achieved by Elon Musk's Neuralink. This company has [00:03:00] successfully implanted a brain chip in a human patient for the first time. This development opens a new chapter in the use of brain computer interfaces, promising to revolutionize how we interact with technology and potentially transform the lives of those with physical disabilities.
Neuralink's first product would be called Telepathy, and initial users would be those who've lost limbs. The Neuralink Implant, a sophisticated device involving a transmitter and ultra fine threads, records neural activity and translates it into digital commands. The chip would, quote, grant people the ability to control a computer cursor or keyboard using their thoughts alone.
You can imagine the possibilities here, controlling phones, computers, and all other devices purely with your thoughts. But, with all cutting edge technology, this comes with its own share of challenges and ethical considerations, from the surgical precision required for implantation to implications of reading.
Thoughts, there's a lot to unpack [00:04:00] here. Amith I feel like we are AI optimists on this podcast I do feel like the brain chip part is a little bit on the scary side of just what's possible with technology in the future.
I'm wondering why you think this news is exciting. And then on the other hand, if you're maybe a bit worried about it as well.
Amith Nagarajan: Well, I think I probably am both. You know, I look at it as the next natural evolution of where this technology is heading. So we've talked a lot about AI on the 1st 14 episodes of this pod. And here we're gonna be talking about AI again, because, of course, AI powers a lot of the capabilities of Neuralink. What we're doing here is creating a brain computer interface. So directly connecting a human brain with Everything else in the digital world, which is both very interesting and exciting in the context that they're first deploying it and to help people who've lost limbs or otherwise are unable to control their limbs. Very exciting. What those capabilities, what it could do to help people. But [00:05:00] also there's a whole bunch of other, you know, applications of this, because this is only the first stop for this technology. Neuralink intends to make this essentially a technology that allows people to compete with AI. So part of the thing that people need to understand about Musk is this is one of many companies he runs, and he launched this in large part because he believes that for humanity as a species to survive in a world of AI accelerating rapidly. We need to be able to augment our own intellect and augment our capabilities. And so his long term vision is for these chips to give you, you know, capabilities that are superhuman essentially. And that's where a lot of course, ethical considerations, concerns, questions of if that's even possible, you know, come into play. So, you know, I think it's a super interesting conversation. When I provide executive briefings to associations on AI, I do that fairly regularly to help executive teams just kind of understand where they fit in to the AI [00:06:00] landscape and how they should be thinking about AI in the coming year. One of the slides in that presentation is talking about the growth of other exponential technologies. And in the area of biology, that's one that I talk about a fair bit and how biology is also on an exponential curve. In terms of cost and capability, very similar to AI in some ways, and here we actually have a direct melding of the two because Neuralink's ability to do what is now possible is due to neural networks. Artificial neural networks basically melding with biological neural networks in the human brain. So, you know, just to make it clear, like what Neuralink is doing now is not controlling computers, keyboards, mouses, etcetera. That's the future vision. Right now it is allowing a person who has lost use of their limbs or lost a limb entirely to control either an artificial limb or eventually reconnect with their natural limbs when they've had severed you know, connections essentially.
So, that's tremendous. That's exciting. But that's just phase one [00:07:00] and that's essentially almost like a one way type of communication where the brain is controlling something. But there's not really any information flowing into the brain. So that would be an interesting kind of next phase of it is, you know, can you really get full value out of that type of implantation?
If it's only one way you're just controlling things versus information flowing into your brain. Like imagine a scenario where you're like, Oh, I wonder what time the Superbowl plays. And then somehow, you know, right? That is pretty creepy, but also kind of interesting at the same time.
Mallory Mejias: Yeah, that is a bit terrifying. It's exciting on the one hand, but like we said, a little bit scary on the other. You mentioned applications for this kind of technology outside of those who have lost limbs. Can you expand on that a little bit? If we had this kind of technology widely available, what kind of world would we be looking at?
What would we be doing with those kinds of chips?
Amith Nagarajan: So the other applications Mallory for Neuralink, I think, and technologies like it, because you know there's going to be others like this, right? [00:08:00] Musk tends to be where I admire him is that he tends to just boldly go into territory that no one has even dared to go before, whether it's reusable rockets or the way he's pushed forward with electric vehicles or in the case of Neuralink or his other company, The Boring Company, which I think is a fun name that is, you know, revolutionizing the way tunnels are built. And that's a real positive thing. I think that very quickly, if there's commercial success, others will follow, which can be good, but let's talk about those applications. He asked about. So imagine an environment where instead of having to connect with my computer through a keyboard through voice or any other interface, I can simply think what I wanted to do. That's the idea behind Neuralink. When we talk about brain computer interface, that's literally what's what we're talking about is directly connecting Your thoughts with with digital technology. So it's kind of frightening, and especially if you don't know who the company is, or, you know, what I want, one of Elon Musk's chips implanted in [00:09:00] my brain.
Probably never, you know, I don't think I'd ever be a candidate for that. But, you know, who knows people that are born right now might think of that is the norm. I don't know. But the capabilities keeping to come back to that is, you know, so if you have that capability where you can directly interface with digital technology, What does that mean? Well, if you think about how long it takes to do stuff just from an efficiency perspective, the limitation for most people were for all of us really is the interface. It's clicking a mouse on a screen. It's typing on a keyboard. And if you can directly interface with the technology, that's, you know, potentially a superpower, you know, especially like, just think about this. Someone who can type it, say, 70 words per minute versus someone who can type it 25 or 30 words per minute, which is probably more like the average. Yeah. That person has a major advantage. The person who types slower isn't necessarily less thoughtful. They're not necessarily less capable. They might have incredible thoughts, but it takes them longer to get their ideas into the computer. Now, typing, of [00:10:00] course, is gonna be one of the great things that we reduce the dependency on because voice interfaces are so good right now. That you're able to just talk to your computer. You know, I use a tool called Otter, for example. Otter. ai. I love this tool. I walk around New Orleans and I talk to myself.
But really, I'm talking to Otter. And it looks like I'm nuts. I'm, you know, walking around the streetcar line in New Orleans and dodging streetcars and dodging New Orleans drivers. And I'm just talking, talking, talking. Because what I'm trying to do is record thoughts and notes and then I will have otter transcribe them, which is good.
But then what otter does that's really nice is it has a really good summarization capability, which I can then take and then edit and then utilize it for whatever I want. So it is, it is my thought process, but it's as fast as I can speak, which is considerably faster than I can type. I'm pretty fast at it, but I can speak way faster than I can type. And these technologies keep getting better. So coming back to your question, brain computer interface is kind of that next leap in efficiency between thought to device essentially. And there's a lot of [00:11:00] applications for it, right? It's a general purpose technology, but there's a lot of apps for it. I think what's exciting is if we see this roll out to help people in need who have debilitating conditions, it could be a game changer, completely changes, these these folks lives. I don't know what it means beyond that. There's lots of medical questions. There's lots of safety questions. There's lots of ethics questions. But it's, it's a technology and it's going to move forward at a rapid pace because that's just what these technologies are going to do.
Mallory Mejias: In researching this topic, I found that I believe one of the monkeys that they implanted this chip in last year died when they were trying to have it play a ping pong game. So there are definitely some concerns here with safety and obviously the medical limitations that we don't even fully understand, but also the ethical concerns as well.
Amith Nagarajan: I think one of the biggest concerns, that's clearly the biggest one, is, is, is it safe to use? Is it effective? Is it safe? And those are things that obviously the FDA's processes for clinical trials and so forth, which would be far beyond this first, you know, [00:12:00] test. At scale, right, would be very important to make sure that it makes sense.
And it's obviously a safe thing to do. But some of the other ethical considerations are things like data. So in the era of cloud based computing and then AI on top of it, people are rightfully very thoughtful and concerned in some cases about data. So if this chip has access to your brain and it therefore potentially could access your memories, if that's something the chip could do at some point, that becomes a concern, which you really want a chip to be able to access your long term memory bank. I don't know if that's possible or not, but theoretically right with the kind of advancements we're talking about, it could be. And so would you want that kind of device implanted in there? I would certainly not be comfortable with that personally, but I know that some people would be right. There's some people are like, yeah, whatever, you know, and these are the same people who probably take their corporate database and dump it into chat GPT just to see what happens. Um. Again, you know, there's people along the spectrum at every level, but I think there's, there's a lot of [00:13:00] interesting questions here. This is still super, super early though.
Mallory Mejias: Wow, I didn't even think about the long term memory piece, that's, not that that's possible right now, but that's true, just thinking about that as being a possibility, it's really scary, so Amith, for the record, you would not have a brain chip at this point.
Amith Nagarajan: Hell no.
I don't think I'm ever going to do it because, You know, the idea that that concept is just so incredibly foreign to me. I have no personal interest whatsoever in it. I think it's, I think it's fascinating though. I think that it could be incredibly important in a lot of domains. I also think about it and say, okay, well, where is this technology going to go? So think about parts of the world where people don't have the ability to choose what they do and what they don't do. Think about militaries and think about in, you know, despotic regimes where, you know, basically these people have no choice. And so if these chips are a thing that work, that it gave people better cognitive ability, better reasoning, better whatever. Access to information, right, can mean life or death in a military scenario. So. [00:14:00] This stuff is going to be out there, and I don't actually think the technology itself that is built into Neuralink is necessarily that hard to replicate. That's the thing about the era we live in right now, is it's, there's so much compounding, exponential growth in AI, in compute, in miniaturization of things, and that knowledge base is out there, so. Other companies are working on this. Other companies will be successful with it. And the implications are broad.
Mallory Mejias: We often talk about the idea of it being more of a discovery problem or an engineering problem on this podcast, would you say at this point, It's more of a scientific discovery problem that we just don't fully understand how this works just yet.
Amith Nagarajan: Yeah, I mean, this is way beyond my area of, of expertise. So I would certainly think that it is, but we'd have to lean on someone who actually is an expert in this field. But it certainly seems to be early days. You know, I think of this as like early days of the microchip when, you know, you were really excited just that you could have a screen light up and have Really basic input and output with a computer. That's kind of where we're at with [00:15:00] Neuralink right now. Really basic, like gross motor skill kind of stuff. It's really not super, super refined, but again, we're on an exponential curve with all the underlying technology. So you shouldn't expect anything different here. AI is on a six month doubling curve. And, you know, a lot of other domains are seeing similar things. So I'm excited about it. I'm also really concerned about it at the same time, but I think it's important for folks to contemplate what this means.
Mallory Mejias: Speaking of concern, we will be diving into topic two, which is generative AI and privacy risks. So last week was data week, and we found a really interesting read about a study conducted by Cisco that revealed that 27 percent of organizations have temporarily banned the use of generative AI due to security and privacy concerns.
These concerns stem from potential risks, including the loss of intellectual property and the unauthorized sharing of sensitive information. The findings are based on interviews with 2, 600 security and privacy professionals across 12 [00:16:00] countries. Now, most of these organizations have established controls with 63 percent limiting data entry and 61 percent restricting AI tool usage.
But despite these measures, something that's interesting is many admit. to inputting sensitive datAInto AI applications, including internal processes, employee details and customer information. This has obviously raised significant concerns with 92 percent of the respondents seeing generative AI as a unique challenge, needing a new risk management.
technique. The top worries were potential legal and intellectual property rights, violations, unauthorized information sharing, and the accuracy of AI generated content. Now, something that's also interesting from this study is that nearly all of the respondents, 94 percent of the security and privacy professionals interviewed said customers would not buy from their organization if they did not protect data properly.
Amith, we often talk about the first. AI actions that we recommend taking would be education [00:17:00] on one hand and then some sort of guidelines or policy around that. We talk a lot about the education part, but I'm hoping you can expand a little bit more on the policy or guideline piece.
Amith Nagarajan: Sure. Well, they're, they're both critical with people, if they don't know what they're doing, that is a big risk by itself in a lot of ways. And that's true with AI. It's true with data. It's true with cybersecurity. So that, that's definitely an important point to always reinforce with respect to guidelines.
I think it's really important to have some. So that's the first statement I'll make, is that if you have no guidelines, then people will fall into one of two camps. The do whatever they want camp, or the do nothing camp. And they're both bad. So, your job as an organization is to put out some guidelines, and just outline what you expect people to do. On the Sidecar community, we actually have some great examples of community shared guideline resources that are posted in the AI for Associations. Group and I'd recommend folks check that out. But the point I'd make is [00:18:00] it's not a one size fits all kind of thing. So don't just grab one of the examples that's on that community site and just implemented. You have to read through these examples and think about, like, what are the considerations these organizations went through? What kinds of data are they dealing with? Are they dealing with personally identifiable information, sensitive data like social security numbers, credit card numbers, perhaps health information, and depending on the nature of the organization, they might have different requirements based on on what they're doing. So I think you have to put in a little bit of effort here. Now, one thing that's really important in your guidelines is to establish this idea of a sandbox, and most guidelines do not do this. So the idea behind the sandbox is to say, Listen, we're gonna create a safe space Where you can, in fact, do just about anything. Perhaps even provide some sample data that you can provide. That's been cleansed, de identified, which is pretty easy to do what using AI actually you can. You can identify a lot of data really easily. That's a whole nother topic, but it's possible to create a sandbox for [00:19:00] people saying, Hey, here's some sample data, some sample documents, things that are essentially public domain, right?
Things that don't really matter from a public disclosure perspective and encourage your people to experiment In that sandbox, that's part of what's missing because people will say, well, you want me to learn AI, but you kind of lock things down a bit with the guidelines. You don't want me to do A, B, C and D.
And those are the four most exciting use cases for my job as a marketing manager or a membership coordinator. Then what am I really supposed to do with this? And that's where the do anything or do nothing camps kind of come into play even if you have guidelines. So I think guidelines are indeed very important.
Yeah. I think that putting guidelines out there that are permissive and encouraging around education are really important. And I think you also need to establish what you definitively do not want people to do. So, to give you an example we don't want people using tools that are not licensed by the organization. In conjunction with your private [00:20:00] data. I'll repeat that just to make it clear. You don't want to use tools that don't have an organizational license where you've actually evaluated the vendor. And then you know, use those unlicensed tools essentially or consumer grade tools with your key data. Now, I make that distinction from just using your key data with AI, because it's very important that you understand that AI is just like any other SaaS application. If you go to open AI as a free customer and you put in a bunch of your data, you have essentially no safeguards. But if you are a paying customer, particularly if you use their team's edition or if you use their enterprise product, you absolutely have protections around your data. Open a eyes. Terms of service says they will not train models on your data.
They obviously will not disclose your data to anyone else. Some people assume that just because it's AI means that you can't use your data with it, and that's a false assumption. So it's about the companies you work with, you know, those same people, by the way, who say thou [00:21:00] shalt not use our data with open AI, chat, GPT, et cetera, oftentimes are totally cool with going to a zoom meeting like the one we're on right now to record this podcast and random people show up with random note takers. And these no taker tools like meet geek or one is one example. You don't know where they're coming from and they're capturing probably pretty sensitive information. So you have to be thoughtful about that. Now, why do I hammer home? Think about the vendors carefully. If you have these random tools popping up, You know, on the one hand, you want to encourage creativity and curiosity.
That's what the sandbox is for, but for adoption at scale and to incorporate your sensitive information, whether it's documents or structured data, you have to know who you're dealing with. Just like you wouldn't just go to some random cloud provider and say, Hey, we're going to leave Microsoft and we're going to go to some random office type tool provider and not ever think about where they store your data or what they do with it. So in many ways, it's common sense [00:22:00] that you'd apply to any Sass or cloud based product, but a lot of people like kind of suspend that thought process when it comes to AI thinking that somehow some way AI is different and that the AI models will like automatically gobble up your data just because they're used.
And that's just not how these models work. By virtue of your data, for example, going to chat GPT. If you're on a licensed version, a paid version nothing is going into the model that it retains. So, you know, you're in pretty good shape. So I think the guidelines need to be balanced. But the last thing I'd say is, you need to actually have some education before you do your guidelines.
So if you, like, try to create guidelines, I just told you a whole bunch of stuff for the last couple minutes that, you know, if you didn't know that, you couldn't create good guidelines because you're afraid of the wrong things. So, you know, we have resources, like I mentioned, but creating guidelines is really important to do, perhaps, after you've had just a touch of education. So you have a little bit of awareness and what makes sense to focus on.
Mallory Mejias: Is the distinction here between a [00:23:00] paid individual account and let's say a free individual account because you mentioned the term organization wide license or something like that. Are you saying we should really only use tools if we have like a ChatGPT Teams account, for example, or are you just saying The paid account is safer than the free account.
Amith Nagarajan: Well, the old adage is if you are not paying for the product, you're not the customer, you're the product. That's mostly true, of course, in the world of social media, where you don't pay for a Facebook account or an Instagram account, but that's because you're actually the product that they're selling to advertisers, right? So a similar mindset can be applied pragmatically to AI tools, is that if it's a free tool, You know, what's the business model? How are they going to make money? And what are the terms of service? And what teeth do you have if you're not actually a paying customer? If you have just a consumer grade free account or even a consumer grade paid account, it's typically a different set of terms than if you have an enterprise level relationship. Now at the same time, you know, it takes time to make a decision to say, [00:24:00] Hey, we wanna license chat GPT for the enterprise. It's expensive and it takes time to evaluate. So that's again, where this idea of a sandbox comes in, where you might say, Hey, there's this really cool new video tool called Hey gen, we want to use it for real time video translation.
Super interesting. Do we wanna deploy it for every one of our employees right away? Probably not. We want to experiment with it a little bit. Do we really know much about the company and where they store our data and what their terms of service are? Probably not. At first, let's see if the product's worth anything first evaluated in this sandbox.
And then, okay, now if we're gonna really deploy it at production level, let's do a little bit of diligence. I'm not saying do the same amount of diligence you do when picking an AMS or something like that, but just do a little bit of diligence to figure out who the company is, where your datAIs going to be stored, and then you can make an authorized application for everyone.
Mallory Mejias: That's a great point. Okay. I was saying if we are relying on these enterprise licenses for tools, it would be really hard to encourage experimentation with Munch, for example, which we've talked about, or Hagen, for example, where you don't need your whole team involved. But [00:25:00] I like the idea of the sandbox and experimenting safely before maybe rolling that out to everyone else.
Amith, would you put a pause on the experimentation piece until you have a policy on it?
Amith Nagarajan: I think that your pause would be about as effective as if the world said we should pause AI for six months. Because the reality is, is that people who are motivated to go use the stuff for the right and the wrong reasons, they're just gonna go do what they're gonna go do. Now, most people are motivated for the right reasons, and they want to work with you, they want to work within the rules that you set up for the organization. But if you have none, then, you know, it's hard for them to know what to do and what not to do. I'm not saying you need six months to figure out what your guidelines should be. AI whatsoever as a team, Spend a little bit of time learning about AI educate yourself and within 2 to 4 weeks, put some guidelines in place. I would not put a pause button out there, though, because it's not practical. People are gonna do what they're gonna do. And then let's just even hypothetically say that your pause button [00:26:00] actually worked and you have 100 employees and you paused all of them, and they're not doing anything with AI. that better in terms of risk profile? To actually pause your organization and freeze yourself in time relative to what's happening in the world around you. I think that's extremely dangerous. I think that you're, you know, every day that you go without any appreciable learning for AI in your organization is a day that you become more obsolete. So to me, it's urgent that you learn this stuff. But again, the good news is it's not an all or nothing thing. You don't have to like just throw open the gates and say, throw caution to the wind, forget about all the worries. But I think the pause button on the other end of it is the extreme opposite of what people should do.
Mallory Mejias: Earlier, Amith, you mentioned these AI executive briefings that you do. And earlier this week, actually, I had the opportunity to attend one. And we visited an association that deals with a lot of sensitive information about the, the members and the customers that they interact with. And so they talked about, you know, wanting to have maybe not a pause necessarily, but [00:27:00] wanting to slow down on it to make sure that their hundred plus staffers did not.
accidentally put highly sensitive information into these tools. So I guess it's a balance, but what I'm hearing you say is that these guidelines, you could spin up a version of them pretty quickly and then maybe adapt them as you go.
Amith Nagarajan: Yeah, 100%. I mean, again, we have examples in our community site, which is community. sidecarglobal. com. We'll put that link in the show notes. But, you know, the idea is, is that you don't need to spend months Coming up with guidelines, come out with them, educate people what they are and say, listen, we're looking for feedback.
This is our first cut at it. We're going to keep on improving it. And again, the idea like think about this, you know, all of your sensitive datAIs probably in either Microsoft or Google. So most associations I know are Microsoft shops. They have excel and word and power point and just files and share point in one drive. And if you're in the Google world, it's the same thing. All of your sensitive datAIs in those environments. You might use net suite or Or sage or one of these other accounting providers for some [00:28:00] sensitive data, your A. M. S. Providers probably hosted. You are trusting a lot of vendors with your most sensitive data. So I think there's this there's this bias, which is understandable because it's new. But there's this bias against AI vendors saying because it's AI it's somehow unsafe to share the data. The thing you want to look for is an assurance right in the contract that says they will not train a future model with your data. That's the really most important thing, right? Obviously, you have to safeguard your data. They have to have proper cyber security policies in place. All the things any SAS vendor needs to have to secure your sensitive data. But the key is we want to make sure the models cannot train on our data. That's the key.
The models will access your datAIn order to serve you. But you don't want the next generation of the model to be trained on your data. And all the major AI vendors very clearly say this in their terms of service. So I, I think that it's, it's an education thing because people need to understand the distinction between using an AI tool [00:29:00] and consenting to their data being used for training, which is really where the risk is.
Mallory Mejias: Hearing you talk about this, I'm realizing this is a good opportunity for Sidecar maybe to go out there and do some research and pull together a list of tools that have that in their terms, or maybe do not, and share that with the greater association and non profit space.
Amith Nagarajan: That would be fantastic.
Mallory Mejias: In terms of mitigating this risk with AI tools, we've talked about lots of options on this podcast, a few of which I'm thinking now are running an open source model locally opting for these enterprise licenses like chat, UBT teams, or using one of the built in AI tools in either Microsoft or Google, like you mentioned Amith, like co pilot or Google duet, can you think of any other ways to safeguard against this risk?
Amith Nagarajan: I think those are good options. And, you know, running an open source model locally or within your enterprise environment is not for the faint at heart. Right now, it's, it's hard to do that. Most associations are probably not going to run open source models [00:30:00] directly, but I think it is possible to do that.
And if you have, let's say, some really sensitive data that you're just like, you know what? I'm never going to give this data to any AI vendor. Well, but you, you want to take advantage of AI. Say you have healthcare data, for example, or you have financial data. And you just cannot get over that hump where you want to share that in any form with open AI or anthropic or cohere any of these other vendors in their hosted environments.
Well, you could take an instance of Mistral Mistral medium or Mistral seven, you know, and take those into your environment, which could be on premise and in your own data center. If you really wanted to go that far, or it could be in a hosted environment you're comfortable with, like Azure. Okay. Or AWS
s. And you could do a lot with that open source model. So if you're an association, I'd say that has some degree of technical depth, then that's an option. If you're not, then it's probably out of reach for now at any at any scale. But the other two points about using like a licensed version of a major vendor, like a chat, GPT teams or enterprise are using AI in the environment you're [00:31:00] already in, like Google duet or Microsoft Co pilot are really good points, and those certainly keep you safe because they're in the environments that you can trust. But that isn't to say that there aren't vendors out there that you can trust outside of that scope. Just do your diligence, you know? Just to give you an example, you know, the, the team at Betty Bot, which we talk about from time to time on this podcast and for disclosure purposes is one of the companies in our family of businesses. They provide a conversational AI for associations. Associations will train the bot on literally their entire content. So all of their content. And they don't want that content to get out, obviously, and it's in its native form, and they also want to make sure their content isn't shared with other people and all that other stuff, but they're actually hiring Betty bought to train an AI on their content, right? Because they don't want to say to Betty bought. You can't train your model on our content because that's precisely why they're having Betty bought engaged with their association to begin with. So you have to have a level of trust there that okay. So what do you want from Betty?
You want to make sure your content is used [00:32:00] for only you. So check that box. You want to make sure you're dealing with a vendor that is someone you can trust. You obviously have to have your own framework for that decision to make sure that you're comfortable with, with the people and the vendor. And, and the list goes on, right?
And, but you want to make sure that in that case, actually they are training an instance of an AI for you. So there's some nuances to this that are really important. That's more of an enterprise use case. But I think that it really boils down to some of the same principles that I would suggest. If you were selecting an AMs. Or if you were selecting a financial management system, you know, you need to have those basic safeguards in place and really the key additional one with AI make sure the next generation of the model cannot train on your datAIn most cases.
Mallory Mejias: Okay, a tiny, tiny tangent. I realized we mentioned Microsoft Copilot and I'm not sure if we've announced on this podcast yet that we have rolled it out to a few users across the Blue Cypress family. Amith, what are your thoughts on Copilot so far?
Amith Nagarajan: I'm pretty pumped about it. You know, it's, it's early days just like it is with all these tools, but Copilot essentially is GPT 4, which is the same [00:33:00] AI model that powers ChatGPT for paid users, and it has the context of your documents. So, with Copilot the very first day I had access to it, I was about to do a webinar the following day. On a topic around around data, and I had this really nice PowerPoint presentation that our team had created for me to use for that webinar. And I said, Hey, wouldn't it be great if I could just create a word document that summarizes this PowerPoint document? And so I went into word. I opened copilot popped up right away.
I literally just gotten access to it, you know, an hour before that or something. And I just said, Hey, I'm doing a webinar tomorrow. I'd like to create like a little summary document that I can send people if they're interested in what the webinars about. And I just put that as the prompt, and then it said, Oh, are there any documents that you want me to look at?
And I clicked that document, which was in my recent files, and it did a great job. It created a one or two page summary for me, and you can do that which actually PT as well. You're just in an environment outside of Microsoft Word, right? You're in chat. GPT. You could [00:34:00] upload the document. It can do pretty much the same thing because it is, in fact, the same model. The key here is it's where I'm already working. I'm in word all the time. I'm in excel all the time. I'm in powerpoint all the time, right? And so by having the AI in that environment, you're saving steps. And the AI theoretically should have more and more and more knowledge about me and my organization and all of my documents. Now, where a copilot is today, copilot does not have intrinsic knowledge of every document in your entire SharePoint environment. It just does not. It doesn't have that context. Can it do searches across your SharePoint or what's the Microsoft graph, which is knowledge, including SharePoint and a bunch of other sources? Yes, it can theoretically, but it's very limited. So co pilot today is a stunning advance. On the one hand, but it's also the very, very first version of this. And so I would definitely encourage people to try it out. You know, I'm not saying you should pay for everyone in your organization right away, but try it out with a handful of people, test it, and I think you'll be pleased with what it can do in terms [00:35:00] of saving you time. In theory, when CoPilot really does have broader context, it can truly understand everything you've done, then it's gonna be an insanely powerful assistant because then it has that full context awareness. It's not just about convenience. At that point, it's about knowing everything in your organization that you have access to and helping you with that broader and deeper context.
Mallory Mejias: Yep. Like you mentioned, it seems like for the time being, you have to select the files that you want it to reference. I tried to whip up a quick email to you, Amith about the AI Learning Hub, for example, and it was very formal, saying, Dear Amith, I want to tell you about some exciting news. So I could tell it was not referencing all the emails I had ever sent to Amith because that is not how I write to you.
But I think you're right, very, very early stages right now. And I am quite excited for what's to come there.
Amith Nagarajan: It's going to be fun to watch, and you know, like all things with AI, we're a broken record here on this podcast talking about this, but AI is doubling at an insanely fast rate. And when I say doubling, I mean the doubling [00:36:00] of performance relative to price. It's happening roughly every six months, and so whenever anything is growing that fast and the capabilities are growing, the cost is coming down, you're going to see tremendous innovation.
So with CoPilot, with Google's product, with all these other tools, you're going to see an explosion of new features and new capabilities.
Mallory Mejias: In topic three today, we are talking about New York City's AI hiring law. New York City's Local Law 144 came into effect last year and it requires employers using Automated Employment Decision Tools or AEDTs to conduct annual audits for race and gender bias, publish audit results, and notify job applicants of AEDT usage.
However, a study recently published indicates It's ineffectiveness. Out of 391 employers.
only 18 published the required audit reports, and just 13 included necessary transparency notices in job postings. The law allows employers significant discretion in determining the applicability [00:37:00] of these requirements and doesn't mandate action against discriminatory outcomes found in audits.
This leniency has led to limited compliance and effectiveness, impacting the adoption of similar laws. Elsewhere, Jacob Metcalf, a researcher at data and society and one of the study's authors said if a system is rendering a score It should be in scope. It's in scope, period. If you're wondering how Europe is approaching this, and typically we talk about Europe as being one of the leaders in terms of AI policy, the EU's AI Act classifies AI and recruiting as high risk, requiring rigorous review.
So, despite its limited effectiveness, LL 144 is seen as a step toward better regulation, but I think it's a prime example of us trying our best, seeing what sticks, seeing what works and what does not in terms of AI policy. Amith, what do you think are some, some lessons that we can learn from a law like this?
Amith Nagarajan: Well, when it comes to New York the New York law you're describing, as well as the [00:38:00] AI act in Europe. What's happening there? I'm both critical and complimentary at the same time. I think it's important that government moves on AI regulation and comes up with sensible frameworks. I don't have the answers for what that should be really, but I think it's important that we start now. I also think that you don't that it's important that you don't, like, choke out the industry in your area. So are you creating a competitive environment that's worse for you in your region? So a lot of people in Europe are concerned that the AI regulatory environment that's being constructed there is going to stomp out innovation and cause AI companies like Mistral, who's based in Paris. To say we shouldn't be here. We can't do our work here. We can't compete if we're based in, in the, in the EU and I think that's an important thing to balance. Right? How, how competitive can you be versus how safe are you now coming to this area of high risk and why New York City decided to put this law into place. I do think that automated reviews around employment decision making is incredibly important to look at carefully, [00:39:00] very, very carefully because, you know, biases exist in both computer systems and with people. And where I'm excited is I actually think computer systems might be able to help us identify biases. You know, more readily than people would be able to on their own. So that's interesting. But the concern here is that the biases of the prior human decision decisions that have been made will negatively influence the AI to repeat those decisions that scale. And so, for example, if certain names, certain types of names, for example, are generally not accepted by a candidate screening system, and that was driven by some biases of prior human reviewers that could negatively influence the way I might score, especially since these AI systems are not necessarily transparent, right?
Some of them can tell you their reasoning a little bit in terms of why they decided a or B. But a lot of times it's just they'll give you a score and they'll say, Hey, it's 42 or 95 right in terms of what what the score is and [00:40:00] with a lack of transparency in terms of how it came to that conclusion. Was there a rubric used, for example, that was used that actually drive that score? You have a big concern. Now, if there was more transparency and interpret ability, which is a topic we've discussed In prior episodes, you will solve a lot of these issues because, you know, AI again, it shouldn't be a magic black box kind of thing that just does something amazing. It needs to be explainable.
And that's the direction a eyes moving in generally. And for a problem like this, actually, I think there's some fairly straightforward technology solutions. If you go to AI and I say, Hey, I'm gonna train in AI right now. And here's what it's gonna do. It's gonna take in 1000 resumes. And and the jobs that they've applied for, and it's just gonna score them, right? I'm just not giving it clear direction. I'm just saying, score these on quality and give me the top 50 people I should go interview. And if I just generically do that, then I don't know what the AI is gonna do. I don't know what it's gonna use.
And whatever biases are baked into its pre training are going to absolutely affect [00:41:00] how that AI scores those candidates. But in comparison, if I have a much more grounded approach where I say, AI system and I build a multi step approach where I say you're going to grade these resumes on the following factors.
Each step is a separate thing. For example, I'm going to grade it on completeness. Were there gaps in the resume that were not explained? Was the resume written? Well, was it formatted? Were there spelling errors? And things like that, right? Like fundamental things. And then, of course, you know, does the education and experience of the individual match the criteria, the technical requirements?
That's actually fairly easy to do without bias. Like if you require a four year degree. Does the resume indicate a four year degree? If you require a particular number of years of experience in particular fields, does that person have that? AI can totally figure that out. So if it's more black box ish, where it's like, just, is this resume good or bad, or given a scale of 1 to 10 or 1 to 100, you can run into a lot of problems.
And that's the kind of concern, I think. The regulators are most focused on. But I think if you build a system that is [00:42:00] designed to score on like certain metrics or essentially like form a rubric around the evaluation, you can eliminate a lot of those concerns. So my point of view is from the tech side, we can totally solve these concerns and actually highlight biases where they exist. But I do think it's important that people step forward and start creating some structure from a government perspective around these issues because It is quite likely that there will be problems, right, from, from these kinds of automated tools,
Mallory Mejias: Have you worked with any of these tools, Amith, before? Or do you have experience with them?
Amith Nagarajan: Across a variety of companies over a long period of time, I've worked with tons of recruiting tools, and actually what we're talking about here is nothing new. AI tools, and even pre AI and AI in quotes, have been in use in recruiting tools for years. And they do things like resume scoring. So that's not new.
What's possible now, though, is to actually almost eliminate the first step in the interview process, the screening process, where, you know, for example, you could say, Hey, I'd like to have the [00:43:00] candidate submit a video explaining why they're a great fit for this position. And no human is ever going to watch those videos.
We're just going to have an AI model rank those videos. Right. That wasn't possible until recently. It's totally possible. Now is that a good idea or not? It's a great question, right? And I don't have the answer to that question, but I think that the key to it is the transparency or interpretability of what the AI model is doing so that you don't just get a yes or no, but you get a clear breakdown of what the issue is. And then going back to New York's law,
they're looking for audit. so
they're saying, Hey, let's look at the actual results. Let's require some transparency in public reporting and let's look for issues, right? Let's all work on this together. If there are issues, let's try to figure out what they are and how to solve for those in the system. Um, the low enforceability of this particular law that you describe is a concern. If, if a regulation has no teeth to it, then, you know, will people pay attention? Generally, I think the answer is no. But I think this is
a great topic to highlight because it also [00:44:00] relates to a lot of associations have to be concerned with. With their own processes
and policies, whether it's government regulated or not, um, isn't really the issue necessarily for the association to consider. It's more of, hey, we're going to evaluate 500 different proposals for speakers for our upcoming annual conference. How do we do that process and mitigate negative biases? So those are similar issues. I think that's where this kind of carries over into the associations. You know, daily work as opposed to most associations I know aren't hiring thousands of people or hundreds of people like a lot of commercial employers do.
Mallory Mejias: With these recruiting tools, I imagine in the past, and maybe even still today, they were scanning resumes, like you mentioned, for things like numbers, certain keywords, certain skills, certain competencies. I'm wondering, and maybe you don't know this, but, Do you think nowadays these tools are capable of being more creative, quote unquote, and when I say that, I mean, there are sometimes you might get an applicant in that doesn't have the exact skill [00:45:00] set that you want, or maybe has had some jobs in different industries, but if you think through, okay, well, if they've done that in this industry, that could be applicable to the job that they're applying for today.
Do you think these tools are capable of that kind of Bridging or stretching,
Amith Nagarajan: Definitely. I mean, language models, video models, audio models, they're all capable of bridging across language. And there's nuance and there's a lot of things in language and communication. That, These tools are very good at identifying categorizing, ranking potentially. So, I haven't personally built a system like this at this point.
I think that, you know, there's abundant opportunity to do it. But I, I would tell you that the primitive building blocks, right? What I'd call the Lego blocks of the AI world are there. There are language components that are out there that can very effectively. Understand the difference between, you know, something that's sarcastic and something that's not right.
Or if you have someone that has a really creative video that they submitted versus someone who's like, you know, Super stiff. [00:46:00] You know, that's a difference that AI can 100 percent pick up on. It's not just the transcription of the words that are being said, but it's the tone and it's the delivery. And for certain positions, verbal communications are super important.
And so could you use that as a screening technique? If you're hiring, say, for example, salespeople or whatever the role is that requires, you know, a good, a good skill set there, you could, you could definitely do that. And that's, that is, that's going back to the science versus engineering Question earlier here.
It's more engineering than science. The tools are there. The building blocks are there to do these kinds of things
Mallory Mejias: and you touched on this briefly the idea that, of course, all humans have bias inherently, and we're creating these tools. So it makes sense that these tools have bias at this point. But you were saying you believe we'll see a world where maybe these AI tools can help us with our own bias, identifying it and maybe be less biased than we are.
How would that work in the sense of creating these tools? How? How could we create tools without bias if we are biased?
Amith Nagarajan: in a really short way. [00:47:00] I describe it as we need diversity in our training data. So if you train in one way, so let's say you take an AI and train it on one chunk of content that's world class, wonderful, great content. But I have a different AI. It's the same exact model, right? It's the same algorithm, but I train it on a completely different data set. The biases reflected by those two AI models once they've been trained will be different because they've been trained on different data to give you another example. Let's say that if you had an identical twin and that person was separated at birth, kind of like in the Arnold Schwarzenegger, Danny DeVito movie twins, right?
And one went to, of course, I don't think they were reportedly genetic twins. But the point is, is that if you had identical twins and you separated at birth and one person was educated, let's say in China, And the other person was educated in the United States and the person in China and the person United States have literally the same DNA and let's say they were both given the same kinds of nurturing, both in great [00:48:00] environments, both given great nutrition and one was trained and educated on Chinese curriculum and another one was trained and educated on American curriculum.
Let's just say both an equally compelling, great universities, blah, blah, blah, right? You're going to have a very different point of view from the person. Who is trained
So going back to diversity, if you have multiple models and you work them together, you orchestrate multiple models talking to each other, they can check each other's work.
So imagine an evaluation done by model number one that was trained on a certain set of data and evaluated a candidate. And then model number twos job is to come in and say, Hey, did model number one do a good job? Or was model number one biased in certain ways? How can I categorize the biases that may be evident in the decision making of model number one? So diversity solves a lot of problems for us as humans. And I think it's it's a really incredibly underappreciated aspect of how teamwork works. When the greatest ideas come to mind, it's from people with divergent thinking. [00:49:00] Then working together in unison to solve problems that are at scale beyond what any individual can do.
And the same thing can be true for AI, because the training data and the analogy I'm drawing with university or just the country you grew up in is very similar to how these AI models work. So I think that's a really exciting part of this. It's kind of the opposite side of the coin where people are concerned that AI systems are going to increase bias, and that's a possibility. I think we can use AI to, you know, make the world more equitable.
Mallory Mejias: So is it fair to say in the examples you gave that we are not necessarily eliminating bias, but maybe mixing different biases so much that we're kind of eliminating it in a sense?
Amith Nagarajan: I don't think we're eliminating bias ever. I think we're identifying it
so that we know that we can be comfortable with what those biases are. We will always have biases and it's like, even by definition, the rubric we established that says this is the criteria that we should evaluate someone on is a set of biases.
Are they the right answers to what we should hire for? You know, it's ultimately judgment that's being used and judgment is fundamentally a biased function.
Mallory Mejias: that [00:50:00] makes sense.
There are tons of great AI resources out there for you, many of which are free. You can listen to this podcast, of course, but there are tons of other great AI podcasts out there, free AI courses. And of course, we want to remind you that we have the sidecar AI Learning Hub as well. As a reminder, that is flexible on demand lessons that we regularly update.
You get access to weekly office hours with live experts, and you also get access to a full. flourishing community of fellow AI enthusiasts within the association and non profit space. And on that note, you can sign up as an individual, but you can also sign up your whole team, all the staff at your organization for one flat rate based on your organization's revenue.
We have a few teams that have done that thus far, and we've gotten some great feedback. So if you're interested in learning more about that opportunity, sidecar global. com slash bootcamp. Thanks for your time.
Amith Nagarajan: Thanks for tuning into Sidecar Sync this week. Looking to dive deeper? Download your free copy of [00:51:00] our new book, Ascend, Unlocking the Power of AI for Associations at ascendbook. org. It's packed with insights to power your association's journey with AI. And remember, Sidecar is here with more resources for webinars to boot camps to help you stay ahead in the association world.
We'll catch you in the next episode. Until then, keep learning, keep growing, and keep disrupting.

January 31, 2024