In this episode, Amith and Mallory explore the role of AI in the association world. Focusing on the integration of various data types, Amith highlights how AI can enhance member engagement and operational efficiency. The podcast dives into the different types of data, the practicality of unifying disparate data sources into a Common Data Platform (CDP), and exhaust streams, the byproducts of online interactions and digital activities. This episode provides valuable guidance on harnessing AI for innovative data solutions in associations and nonprofits.
- Let us know what you think about the podcast. Drop your questions or comments in the Sidecar community: https://community.sidecarglobal.com/c/sidecar-sync/
- Join the FREE webinar “Own Your Data, Own Your Future” on January 18, 2024 at 11AM CT/12PM ET https://sidecarglobal.com/data
- Join the AI Learning Hub for Associations: https://sidecarglobal.com/bootcamp
- Download Ascend: Unlocking the Power of AI for Associations: https://sidecarglobal.com/AI
- Join the CEO AI Mastermind Group: https://sidecarglobal.com/association-ceo-mastermind-2024/
Thanks to this episode’s sponsor!
- AI Learning Hub for Associations: https://sidecarglobal.com/bootcamp
Tools/Resources mentioned:
- AI Taxonomies: https://sidecarglobal.com/artificial-intelligence/revolutionize-your-associations-content-taxonomy-with-ai/
- Betty Bot: https://bettybot.ai/
- Descript: https://www.descript.com
- Chat GPT: https://chat.openai.com/
- Gemini: https://deepmind.google/technologies/gemini/#introduction
- Rasa: https://rasa.io/
Social:
- Follow Sidecar on LinkedIn: https://www.linkedin.com/company/sidecar-global
- Amith Nagarajan: https://www.linkedin.com/in/amithnagarajan/
- Mallory Mejias: https://www.linkedin.com/in/mallorymejias/
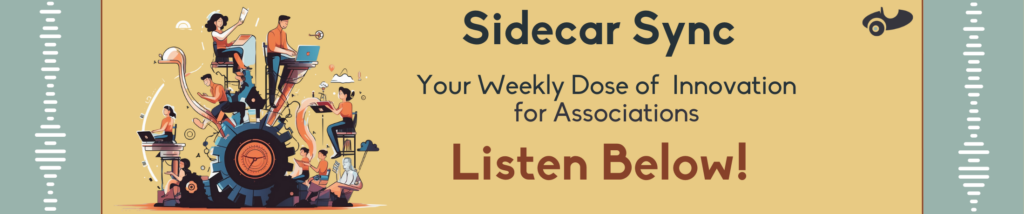
Amith Nagarajan: [00:00:00] And that allowed us to bring all of our data together into a unified simple environment, which we can then do analysis on. We can do AI driven interaction with it. There's a lot we can do with it because we brought the data together from these different structured sources into a common data platform.
Welcome to Sidecar Sync, your weekly dose of innovation. If you're looking for the latest news, insights, and developments in the association world, especially those driven by artificial intelligence, you're in the right place. We cut through the noise to bring you the most relevant updates, with a keen focus on how AI and other emerging technologies are shaping the future.
No fluff, just facts and informed discussions. I'm Amith Nagarajan, Chairman of Blue Cypress, and I'm your host. Greetings everybody. And welcome back to the Sidecar Sync. We are super pumped to get started on this latest episode all about data. And before we get started, here's a word from our sponsor. [00:01:00]
Mallory Mejias: Today our sponsor is the AI Learning Hub for associations and non-profits, if you aren't familiar, the AI Learning Hub offers flexible on demand lessons. So you can learn AI at any time, whenever it fits into your busy schedule. Not only that, but we consistently add new lessons to the bootcamp based on the latest AI advancement. So you can be sure that you're keeping up-to-date with what's going on in the AI space. You also get access to weekly live office hours with AI experts.
So you can ask them all your questions. And finally, maybe the best part you get access to a vibrant community of fellow AI, enthusiasts. So you can connect on your AI journey, share your challenges and learn and grow together. You can get more information on this and the bootcamp itself at sidecarglobal.com/bootcamp. Today is a special episode all about data it seems like we're doing a lot of special episodes lately, but that's because there's a lot of special things going on with AI. Today we are talking about data. Different types of data. What it is. Where to find it and [00:02:00] ultimately how to leverage it. I saw a funny commercial on the TV the other day.
I'm sure a lot of you listeners have heard it as well. Maybe you too, Amith. And it's something along the lines of Matthew McConaughey in a Wild West setting, and he says, if AI is the Wild West, does that make data the new gold mine? I thought that was an interesting commercial, and I feel like you agree with that, Amith Is that right?
Amith Nagarajan: Yeah, a hundred percent. And anything with him in it is usually pretty funny.
Mallory Mejias: And today's episode is also special because we are letting you know about a webinar we have coming up this month on January 18th. It's called own your data, Own your future. Amith can you tell us a little bit about that webinar?
Amith Nagarajan: Yea, love to. So, You know, this episode is dedicated to data. We're going to dig into all sorts of facets of data, different types of data and how data is not only relevant to AI. But such a critical ingredient to your success as an association or nonprofit. And it actually always has been, but more so than [00:03:00] ever.
And this webinar coming up next week on January 18th is about the idea of truly owning your data. Now what we mean by that is that data is owned by you, truly owned by you, only when you have complete control over it. You might legally own the data that is in your community. You might legally own the data that is in your CRM because the contract you have with the vendors for those SAS solutions says you own the data.
But as a practical matter, those data sets don't exist in a standard format that allows you to access them anytime you want. completely and interact with the rest of your data. And that's a big, big problem when it comes to your AI Strategy. Data is the fuel for AI. It's the gold. It's the thing you need in order to drive any degree of sophistication with your AI. And until you get your data into an environment and a format that works well together completely and [00:04:00] seamlessly, you have a major liability. And that's what the webinar is about. It's about framing the problem, explaining the background. And then talking about solutions to this problem. The good news is there are solutions and they're within reach. So we'll be talking all about that on January 18th.
Mallory Mejias: This webinar is free for anyone to attend. Again, it's on January 18th, 2024 at 11am central, 12pm eastern. You can sign up for this webinar at sidecarglobal.com/data. And we'll also be linking that webinar in the show notes. This episode's a little bit different because in the past we've done timely news updates.
Well, today we're diving into different topics within the idea of data. But first, I feel like we have to start off with a pre topic question, Amith What is data?
Amith Nagarajan: It's a great question. Data can literally be almost anything.
You know, and data and information are two different terms. We'll dig into that as we go through the episode. You know what constitutes information versus data were actually kind [00:05:00] of simplifying it. this conversation by using data to somewhat mean both.
But we'll dig into with the difference between the two because information and data are not the same thing. But data essentially, at its most fundamental level, is something that you store something that provides you insight. It could be text. It could be images. It could be what we call exhaust streams from other applications.
There's a lot of different things that constitute data. It’s not just the data in your AMS. or LMS. Those are the places people tend to go in their mind. When you say, where's your data? It's also an Excel spreadsheets. It's also in text files. It's also in your email. There's so many different forms of data.
Some of it is primary data where it's things that you actually work on both collecting the data and then working with the data, analyzing it, smoothing it, all those kinds of things. And there's other data sets that are secondary that are essentially like a byproduct of the business process. For example, keeping track of all of the processes around abstract submission for a [00:06:00] conference.
The byproduct of that is, you know, the approval and rejection status of those abstracts. And that's an example of a byproduct or what we like to call an exhaust stream from business process. So we'll be talking all about that. But data is literally everywhere, and it's exploding in volume, and it's really, really important for you to have access to your data.
Mallory Mejias: Absolutely. So data is something that you store. It is something that provides you insights. And in today's episode, we will be talking about text data, specifically visual and audio data. We'll be talking about structured data. And finally, we’ll be talking about those exhaust streams that Amith just mentioned.
But first, let's start with something that we all know and recognize. Text data is everywhere. From the flurry of tweets on social media to the extensive archives of academic journals, at its core, text data encompasses a wide range of written content, such as social media posts, emails, business reports, and online articles.
This richness makes text data a gold mine for AI [00:07:00] applications. With the rise of advanced natural language processing techniques, particularly in language models, AI can now delve into this ocean of words to extract meaningful insights. Automate responses and even generate new content for associations and nonprofits leveraging text data can transform how you engage with members, analyze feedback, and stay informed about industry trends through AI tools, like sentiment analysis, chat bots, and content summarizers text data becomes a powerful asset in enhancing communication strategies would you say text data is one of the most abundant forms of data? Honestly in the world, but in an association and nonprofit as well.
Amith Nagarajan: It certainly is in terms of volumes, because that's the primary way we've communicated for a number of years through technology. Of course, the amount of information contained in text data is a lot smaller than the amount of information contained in an image or a video. So the information content of the information density and text data is [00:08:00] relatively low, but the text data itself is highly abundant, as you said.
We have it everywhere. We have it in emails. We have it in obviously web pages. We have it in chat conversations. It's literally everywhere that you look.
Mallory Mejias: Can you elaborate a little bit more on the difference between information and volume?
Amith Nagarajan: I'm happy to. So when we talk about information, we're talking about, you know, essentially what is in that data stream. So if I give you a text document and let's say it has 1000 words in it, how much information is in there? How dense is the information in that Document. And if in comparison, if I give you an image, how much information is in that image?
Now, there's lots of ways of kind of theorizing about how to describe the information density of data. But a really simple way to do it is actually put that file, that text file. into a compression tool, like creating a zip file. If you ever create like an archive or a zip file, you'll notice that text [00:09:00] data is highly compressible.
So mathematically, it's possible to bring that file, that text file down to a very tiny size. You might have like A one megabyte text file that ends up getting zipped down to 10 or 30 or 50 kilobytes, you know, a factor of 5 to 10 percent of its original size. And the reason that's possible is because mathematically you can actually represent the information in that text in a much smaller compact package. That's what compression is all about.
And then image data. On the other hand, if you take an image and then try to compress it, generally you have a much lower rate because there's much more information in the image. That's one way to think about it. And the same thing is true for video.
And there's a lot more nuance to that conversation, but a very simplistic level. You can think of this this way. So imagine that you are watching Mallory And me our podcast through our soon to be launched YouTube channel where we're doing video as well as audio.
So watching us speak, [00:10:00] particularly if we have some visual aids, is one level of information. Now, if we just took the audio out of that and said, okay, we're only going to use the audio, we're going to put it on Spotify and Apple podcasts and so forth. That's still a lot of information. It's all, it's all of the audio, but you lose the visual.
And particularly if it's just the two of us talking, it's not that much information loss. But if there was like a demo on the screen, or if there was some visual aids, some slides we put up on the video podcast, then that would, of course, be significant information loss.
And then finally, if you take the Audio and said, Hey, here's the podcast. Let's create a transcript. A text based transcript. That's even less information because think about it. If you read the words that I am speaking to you now, you don't hear my voice. You don't hear my tone. You don't hear my inflection. You don't hear the volume changes that I make as a speaker. You're losing information.
So the words might be the same between the transcript and the audio and the video. But the amount of information density is higher in other mediums. That's why I like to say that [00:11:00] text is abundant. It's But it has a fairly low information density, if That makes sense.
Mallory Mejias: That actually makes a lot of sense. That was a really helpful analogy there.
I think we could all agree saying that text is one of the most abundant forms of data out there, if not the most abundant, that mission driven organizations like the examples we gave have lots of it.
How would you, Amith, recommend that they begin to leverage the text data that they have?
Amith Nagarajan: The number one thing I'm going to be recommending across all of these data types is to start creating and brainstorming an inventory of all the data that you probably have out there. So a lot of people have a pretty narrow view of what data is. Again, as we said earlier, a lot of people immediately go to more structured data forms, which we'll come back to later in the episode. Things like the data in their CRM or AMS, things like member data, information about who attended events.
And of course that's a very important and rich source of, data for your association. But text data does exist in those systems, by the way. A lot of times you'll have [00:12:00] these comment fields inside a CRM or inside an activity record in a CRM where you can capture like comments about the member or about an activity, and that's useful when it's used, but that's an unstructured element of data inside a structured source. But text data tends to be abundant in all of the other ways that we communicate with people.
So clearly email is a massive one. We're always emailing each other internally. We're emailing members. We're emailing vendors. We're emailing partners.
We also have instant messaging communication tools. You might Microsoft Teams as we do here at Blue Cypress. You might use Slack. you might use other communication tools.
And those communication tools are, of course, abundant for text as well.
But then think beyond that. Think about things even like text messages. Think about like apps that you use at your conferences where people are able to communicate with each other in real time.
Your online community is another incredibly important source of real time insight. It might be a small percentage of your members. Most online communities typically don't penetrate more than five or 10 [00:13:00] percent of the membership base at large. But the data in there can be very valuable because those are some of your most engaged, you know, essentially loyal and bought in members.
And so that data is often completely unmined and unused in AI or for anything else for that matter. So text AI is abundant. It's everywhere. But don't think about just the most obvious sources.
Last thing I'll say is your your office document repository. So if you're a Microsoft shop, that would be Microsoft Office, Microsoft Word, Excel, PowerPoint.
Those sources, of course, have a lot of text in them. If you're a Google shop, it's Google Docs, Google Sheets, et cetera.
Mallory Mejias: I think it's unique to think about communities as having lots of text datat's obvious on one hand, but maybe it's not so obvious on the other hand. What would you recommend that associations do with their community text data? What are examples of what they could do with that information?
Amith Nagarajan: Well, the first and simplest thing is to be better listeners. Members don't like it, customers don't like it, [00:14:00] when they feel like the company isn't listening to them. And so, people will want to express both frustration and when they're happy, through a variety of mediums. They might do it on public social channels, they might do it on your online community.
And these tools tend to be pretty simplistic these days. They don't really have good social listening or good listening capabilities, but I guarantee you most online communities and a variety of third party tools will help you get a better sense, through advanced language processing. You know what's going on? How do people feel about you? That kind of sentiment insight that up until recently has been super rudimentary. Like it's essentially, these tools have been scanning for keywords saying Someone said like an expletive or someone said bad things about you, right? And that was that was how simplistic the NLP or natural language processing was until language models really hit the scene.
But now we can really do a much better job of looking at the types of posts that are in our community and even pulling in content from other social platforms, combining that together and getting a much [00:15:00] better understanding. Both in terms of where people are happy and where they're not. But also, wouldn't it be. great if you're actually creating content that people wanted?
You could look at what your people are saying, what they're talking about, and probably get a lot of insights into what your editorial calendar should be.
One of the examples I always like to kind of hammer on with folks is people are still in this mindset of setting an editorial calendar for their journal or even for their newsletter that might be a year in advance.
They might say, hey, our July issue is gonna be our issue on blah blah blah topic. And I don't, I'm not against the idea of outlining your general content strategy. What I'm against is setting that in stone. And because, you know, if, if a topic arises that's extremely important and you're not covering it when your audience wants you to cover it, you seem tone deaf. And that's, unfortunately, a lot of brands, including associations, fall into that trap. So, first thing I would do is deploy various types of social listening algorithms. There's, again, third party tools that are doing this quite nicely.
And I do expect most of the online community software solutions to have tools like that built [00:16:00] in now that AI is not only prevalent, but very easy to incorporate into all of these pieces of software.
Beyond that, though, I would say there's a lot of what we'll talk about later. This idea of exhaust streams where Imagine if I'm talking about a particular topic in the online community, but I haven't yet registered for an event that's coming up that's really related to the topic I'm discussing.
So say, for example, I'm on Sidecar's online community, which is free to the world, by the way, and you posted something saying, I'm wondering how to best prepare my data for AI. And I post that a week ago. Well, wouldn't it be great if the AI could automatically determine that that person might be, a good fit for the January 18th webinar we have coming up all about that topic. Right?
And we had sidecar, by the way, haven't deployed that form of AI Yet we will be, I'm sure. But, you know, the ideA is that would be the type of value add personalization and engagement that people would rave about because they'd say, Oh, my gosh, these folks are really listening to me and what I need.
And you have a lot of that data sitting there in [00:17:00] these types of repositories.
Mallory Mejias: Last year I had the opportunity to attend ASAE Annual. It was a great event in Atlanta. And I spent some time talking with folks there about Betty Bot, which, for those of you who aren't aware, is an AI chatbot built for associations. And we heard a lot of the same things over and over, which were, Oh, we would love to implement something like Betty Bot, but we need to clean up all the information. that we have on our website.
We need to clean up our blogs, our courses, so on and so forth. Do you think that's an essential first step, Amith?
Amith Nagarajan: Only if you want to fail, I think that that's the first step that people historically think of because somehow they think it's this magic solution that will enable them to do great things. And I say that somewhat tongue in cheek, but in all seriousness, though, if you try to clean up your data, you will fail because ultimately it's an, it is an AI scale problem.
It's not a human scale problem. But it's also a moving target. It's the same thing that goes for why taxonomies usually fail. Organizations spend a lot of money and a lot of time and the taxonomy is almost immediately out of date because the field is moving. [00:18:00] Then, maintaining the taxonomy, just, managing all the different tagging for all the different articles they have is nearly impossible.
And there are good solutions for that with AI. by the way.. That's another thing that we've talked about quite extensively in the past with Sidecar content. We'll link to some of that in the show notes as well, in terms of how to do taxonomies with AI. But the point is, is that these are not solvable problems.
Even with AI, the idea of quote unquote cleaning up your data, is really like a false obstacle, in my mind. And one of the reasons for that is, you don't need to clean up the data in order for you to understand the text data. You can look at a document that has all sorts of flaws and still gain the essence of it, understand what it's about. Here's the thing, AI can do that too.
Language models, even stuff that's not state of the art, stuff that we've had for a couple years now, can look at a document, can look at an email and quickly assess the underlying meaning of that document without any cleansing. And so it's an unnecessary step.
I'm not saying it's never appropriate in certain cases, but [00:19:00] generally speaking, to throw that up as a gate you have to pass through is almost a guaranteed, you know, failure path.
Mallory Mejias: I want to dive more into visual and audio data. We know that visual data includes a wide array of content like images from your events, or graphics and educational materials, and videos from your conferences and workshops. Audio data encompasses everything from recorded speeches and discussions to audio tracks of webinars and member feedback and even podcasts.
Associations typically accumulate mountains of these types of data through events and courses, but the challenge lies in effectively utilizing them. We all love to record the sessions at our conferences, but what do you do with them after? And I know this sounds rhetorical, but it's actually a genuine question.
So please leave us some feedback in the sidecar community. We often have this conversation about what to do with session videos and audio. From our digital now conference, for example. But I think this is an interesting opportunity to talk about the sidecar sync podcast itself and how we leverage the audio.
So every episode we [00:20:00] use a tool called descript and AI powered editing tool for video and audio to create transcripts. For the podcast, and you can actually edit the transcript right there. Instead of having to just look at the audio file editor, which is how I imagined it work in previous times, and it allows us to edit our podcast efficiently.
But what we do with the audio, I think is another interesting step. So we actually take chunks of the transcript, the topics that we talk about. We work with chat GPT, and then we create really timely, relevant blog posts. on the topics that we talk about in the podcast. And because we're leveraging AI here, we're able to do this pretty quickly.
In just a couple hours, churn out a few blog posts, going back to the podcast and not only be an entity that's talking about AI news, because surely there are many organizations that are doing this exact thing, but talking about AI news in the context of associations and nonprofit organizations and doing that really quickly.
So on that note, Amith, I'm wondering. So [00:21:00] we, we use this audio data that we have from the podcast and put it into Descript, for example, but to do that, it's creating a transcription of the audio and we're essentially then working with text data. Are there any ways that you can actually use audio data as is, or do we kind of always need to go through that intermediate step of getting it into text first?
Amith Nagarajan: So the models that we're currently working with primarily, including chat GPT, Google's barred, powered now by the Gemini pro product or model. I should say these are all text based. Gemini was trained on both text and other modalities. But as AI, becomes more capable, will need to train AI natively on multiple types of content.
And so the idea that your images and your audio and your video has value even outside of transcription, which is, of course, very powerful use cases you've described,
Is really strong. And some of that actually may be coming as opposed to things you would do with it right now.
So [00:22:00] one comment would be that with your mountains of content with You know, proceedings from conferences in the past webinars, things like that.
You have all this audio and video, and right now you're probably doing very little with it. It might be on your LMS. If you're a more advanced association, you might have done a little bit of post production work and said, Hey, I'm going to trim that. Maybe make compilations of it, create ways to make it easier for people to engage.
But typically that's for a very small fraction of the overall content, you have.
And some of that content's evergreen, meaning that it's not perishable. Some content, of course, is perishable, where if you listen to a podcast, that was from five years ago, and it was about AI well, that would probably not be the best source of information because things have changed so rapidly. But if you listen to a podcast from five years ago about perhaps culture. Or organizational design, those topics might still be quite relevant five years later, maybe not 50 years ago because so much has changed societally and so forth. But, the nature of the decay rate of a piece of content is dependent upon the subject matter.[00:23:00]
And guess what? AI is really good at helping you figure that out. AI can look at your content. And give you a pretty good assessment of what's the life span or the shelf life, I should say, of this asset. So that's one of the things you can start doing is to run your content through an AI and say, Hey, here's the transcript from this video: give us a number of months rating of what you think is an appropriate shelf life. And then start using that to then think about what the future would hold in terms of which, which pieces of content do you keep for longer? Which ones do you promote more? But, you know, coming back to the broader conversation Mallory, I think what you've described and shared with our listeners about what the Sidecar team does with this podcast. is really instructive because it's a multi-faceted output from a single effort. So, you and I spend a little bit of time ahead of this podcast preparing for it throughout the week. We, you know, collate different ideas and topics.
We get together, and we record the podcast. And then from there, there's the post production process, which is editing [00:24:00] and then the process of creating blogs and so forth. And that's something that I think is a pretty easy thing for a lot of folks to start doing with chunks of their content, which might be You know, interesting audio clips from their conference.
But the other thing, too, is we can automate way, way more than what we're currently automating. So going back a few episodes, we talked about AI. Agents, which are this this concept of being able to chain together multiple discrete processes, where, for example, this idea of a process that might edit a podcast that's going to become pretty much a fully automated thing, maybe with human review.
But the idea of editing it, which is What are you looking for? Typically, you're looking for pieces that need to be smoothed over or eliminated. If there was a pause, you're looking for changes that you want to make based upon background noise. The typical things you do. These tools are very good at doing what you tell them to do, but very soon they'll be able to automate the entire process or give you essentially a rough draft of a final, you know, cut would look like.
And then from there, the next set of [00:25:00] steps of, you know, creating the blog topics and then creating the blog posts and actually posting those on your website. Then what about creating little video clips? And what about posting those on social? So there's so much of those steps, which is still manual, and that will all be automated in the very near future.
Probably in the next 12 months, I could see. Many organizations automating that type of workflow. So I digress a little bit in terms of the core topic here about the nature of that data.
But I think there's so much abundant opportunity right now, even with the AI capabilities we already have.
Mallory Mejias: We definitely talked about agents in many previous episodes. I am excited for the day that I can create an agent with little to no code. Amith do you have any insights there on like how far out you think we are from something like that?
Amith Nagarajan: it's, It's feasible right now. You just need a little bit of engineering skill. And so therefore it's technically feasible, but probably out of reach for 80 percent of associations. If you found yourself in a situation where you said, Hey, We have a conference where we have, you know, 20, 000 attendees. We have 700 sessions. We have 40 [00:26:00] keynotes and we have all this great content. We really want to automate this now. It's totally manageable to do that. It would require a little bit of custom development work. You use a framework like auto gen, and there's there's others like auto gen. And you could create a tool that goes through and does exactly what you want. It's not yet point and click. It's not yet something someone, for example, on the marketing team or the meetings team would do without technical assistance.
But that's coming. There are a number of companies working on visual style point and click editors where it's like a workflow tool. It's like a lucid chart or a vizio chart where you can just basically draw diagrams and it'll do what you want it to do. That's coming. In fact, Microsoft's making a big, big play in this area with their tool called Power Automate.
Which is exactly what I described. They're adding a ton of AI capabilities, including a copilot capability directly to power automate. So very exciting time. That's what I'm saying. In the next 12 months, I think this will become feasible for just about everybody to automate many of these business processes.
Mallory Mejias: We paired audio and visual data together because they go hand in hand [00:27:00] in many cases, but I feel like a lot of our conversation thus far has revolved around audio from sessions, let's say, or audio from a podcast. Can you think of any instances where we can isolate visual data on its own and make that useful?
Amith Nagarajan: Sure. I mean, there's tons of scenarios like in scholarly journals, you have tons of tables, charts, graphs, images, particularly in scientific literature of. You know, experiments or specimens or images of whatever, right? Whatever the subject matter is. So there's a lot of still image data as well as video that's out there. And this is extremely information rich. You think about a field like radiology, for example, and those journals are chock full of images of different kinds. And so, it's really, really important to think about that stuff. Particularly when it comes to what I call your gold standard content, which would be, Especially if you have a peer reviewed journal, if you, if you don't have that, you know, even many of your content assets that have gone through some form of review where you consider the content to be highly accurate, highly [00:28:00] respectable, usable as reference material, essentially, and associations have tons of this stuff. So not like a casual blog post that was contributed by some guest author that's never been looked at by anyone. That wouldn't be gold standard content. It's content. It's content. But the content that you really put a lot of energy into, and a lot of that stuff is filled with tables and charts and graphs and images of different kinds, and that's super, super leverageable.
In fact, you mentioned Betty Bot earlier. You know, Betty Bot is doing tons of work with associations in scientific realms where this type of image data or, you know, just graphical data is abundant and is able to interpret that information and use it in the context of formulating, you know, expert answers to questions that are coming in, not just the text surrounding the images.
So it's a really, really powerful modality.
Mallory Mejias: Seems like taking the time to clean up quote unquote your data before doing any of these projects would be kind of just a step in the direction of failure, in your opinion, at least. But then you would say that you should catalog the different types of data that you have [00:29:00] before going forward with trying to leverage it. Does that make sense?
Amith Nagarajan: Yeah, I'm I'm basically saying you should definitely catalog or inventory, you know, the different categories of data that you have brainstorm all the different places where you may have data. Not to say that you think those pieces of data are necessarily valuable, but that you know that they're there.
As far as the cleanup is, I'm not saying that universally data cleanup is a bad idea. There are scenarios where data cleanup is really important. For example, In your CRM if you have duplicate records, that's a really important thing to work on. If you have records that reflect where someone worked five years ago, that's really important to fix.
And there's ways to fix that.
So I'm not suggesting that universally speaking, data cleanup is a bad ideAI'm saying that it's something you have to look at pragmatically and look at as a parallel process with everything else you want to accomplish. Because if you put that as a prerequisite, then you will fail because you will never get to the adoption of AI. If you say You know, our data has to be at some, you know, incredibly high standard that is not sustainable or [00:30:00] achievable long term.
Mallory Mejias: I like that. Data cleanup as a parallel instead of a first step. That makes sense. Moving on to our next topic for the day, we're talking about structured data which might be what a lot of you think about when you hear the word data in the first place. Structured data refers to highly organized information that is readily searchable and storable in databases like CRM systems, registration forms, survey results, and financial records.
These data types, characterized by their orderliness and clarity, provide a solid foundation for analytical tasks. For associations and nonprofits, structured data can offer invaluable insights into member behavior, operational efficiency, and financial management. By effectively utilizing AI, organizations like yours can unlock the potential of structured data, transforming it into strategic decisions and personalized member experiences.
Understanding how to collect, store, and analyze this data is essential for maximizing its utility, especially when combined with AI's power to sift [00:31:00] through and make sense of large data sets. Amith, we all have structured data think that's a safe assumption to say on this podcast, but we don't use it all.
And that's interesting to me. It's something that we all have, and it's so valuable, yet we don't use it. What is the gap there between having it and being able to leverage it?
Amith Nagarajan: One of the issues that people run into is the lack of flexibility that many of these structured systems have. So what will happen, I've seen this for a long, long time. And for those of the listeners that don't know me prior to what I'm doing now, which is focused on helping associations in their journey.
For 20 plus years, I ran a software company that was in the association space providing an AMS solution. So, I've been around a lot of the implementations of structured, you know, structured types of systems like this. And what I've seen over the years is that, where people tend to not utilize the structured data very well, is when it doesn't meet their exact needs.
So let's say that you're a meetings manager and you have [00:32:00] a job that you have to accomplish, which is perhaps you need to invite people to an event, but first you have to segment the data. And you have to segment the data based upon some attribute of information that's not stored. In your database. And for some reason, you know, you're unable to add that additional attribute or field to the database.
And that's a common problem in many structured systems is they're highly inflexible or even if they have flexibility, making changes to their structure is complex and expensive. And so it doesn't happen. And so you're this meetings manager who needs to perform this business task and you can't do it inside the AMS, let's say. So here's what you typically do. You export the data to Excel and then you manipulate it in Excel and maybe you have the other data somewhere else. So if you're a more savvy Excel user, you find a way to join it in. Unfortunately, a lot of people are not that savvy with Excel, so they might manually process a file like that, and then go through their process of whatever they're doing, segmenting, marketing, etc.
And then the problem is that the data then lives in Excel. It's in what I'd call a semi [00:33:00] structured format, where it's not structured with the rigor of a system like a CRM or an AMS. But it is somewhat structured because Excel has rows and columns and you can kind of tell what the datas. It's more structured than text.
But you lose some of that fidelity. You lose some of the information because of the inflexibility of many of the structured systems. And this is what you see. It might start off with a couple of processes like this. But then over time, it's quite common that, you know, you'll have dozens of processes like this.
Where that central system actually doesn't have a lot of the information. Many of the business managers need for membership for marketing for various other areas. So that's one of the big problems.
The other problem, of course, is that these structured data sources most of the time are closed systems.
And what that means is they might very well have an API. They might very well even let you access their database in some form or fashion. But most of the time, what you're able to actually get at is a limited subset of the data.
So it's hard to get the data out. [00:34:00] So, you know, even if you use a modern contemporary CRM, like a sales force or something like that, you have to go through an API. The API does have connectors to many other systems, but you're looking at a narrow window into your data. You're not looking at the actual data itself, and that makes it somewhat difficult to access that structure data and use it for a variety of things, including pre-AI. Just basic
analytics. But then, of course, all the AI Things that we want to do.
Mallory Mejias: So then you would say that this gap between having something so valuable and not fully leveraging it would be in flexibility of the tools more or less?
Amith Nagarajan: That's definitely a big part of it. I'd also say the other thing is folks are overwhelmed. You know, everybody's busy. Everybody's working really, really hard just do their job day to day. And I empathize deeply with that. I know talking to so many association leaders and, like staff over the years, that they're, they're like, Yeah, I really want to do this analysis.
And maybe they do have the tool to do the analysis. Maybe they do have the tool to, you know, do a little bit better job on their marketing, and they know kind of how to do it, [00:35:00] but they don't have time to do it because they're just spending all their time, you know, going through the motions of processing things and doing really rudimentary tasks.
And so, that's actually one of the things I'm most optimistic about with AI. A lot of folks are saying, well, AI is going to take my job away. And I'm not suggesting that that's not possible, and certainly in some cases, I think what's more likely to happen in organizations that are financially healthy is to say, Hey, listen, we've got a dozen people in our member services department, and we now only need two of them to actually keep doing the basic member services work that they've mostly been doing because of AI. Automation and You know, automated communication and all this great stuff. What do we do with the other 10 people? Maybe they can start to step up and give them some additional training to do some of these higher order things. And I'm not Pollyannaish to think that everyone can be retrained in that way.
There will be some challenges, you know, in that context. But, the bottom line is, is that it's AI mixture of things. It's definitely inflexibility in the tool. It's a lack of data in those environments because of this, you know, essentially what I was describing earlier, where people start storing bits [00:36:00] and pieces of data in other places because they can't store them effectively in that central system.
And then ultimately also just time. So those are some factors that I've seen over the years that prevent people from really leveraging their structured data in a meaningful way. Oh, and by the way, the other thing that's a big deal is the lack of integration between systems. You might have a CRM of course you do an AMS CRM, something like that. You have an LMS probably for your learning management. You have your online community, which has a lot of structured data. You have a variety of systems, of course, your financial management system. And these things In theory, people will say they've integrated, you know, in quotes, they've checked the box.
And what that basically means is the most rudimentary connection between these systems, where, for example, I've pushed financial transactions from my AMS to my FMS. And that's it. I've pushed basic learning track information from my LMS to my AMS. And that's better than nothing, of course. But there's worlds of information in each of these systems.
And so, people tend to not really do what we're talking about here because they have these islands of data in these [00:37:00] different structured systems.
Mallory Mejias: I can definitely relate to that. And I'm sure a lot of marketers listening to this podcast can relate as well. I have built many a Zapier integration, which is very basic to have data pushed from one place to another.
And although it's, it's a temporary solution, I realized that it's not completely comprehensive.
I think an interesting part of this conversation is around. People having to dig for insights as opposed to insights being presented to people without asking. And that is kind of what I hope to see in the future with AI and data. Last year, I ran a little experiment on my end to see if I could use deal records from HubSpot, which is the CRM that we use to create an ICP, an ideal customer profile.
And I did, you know, I cleansed the data, I mean, manipulated it, so I didn't put anything sensitive into ChatGPT's data analysis feature. but I essentially created fake data, put it in, and it did create ICPs really easily using the deal record information that we had. And this was great, yes, but the thing is, if I hadn't thought [00:38:00] about this with a coworker, we were brainstorming, trying to be creative for uses of ChatGPT, we never would have realized this.
And so, I guess that's where my frustration is. There's so many insights, but we are responsible for digging them up. Amith, do you see AI as being a tool in the future that just gives you these insights without you having to go claw at them?
Amith Nagarajan: I think absolutely it will. And I think you could use it today just with asking a different question, almost which if you zoom out and say, Well, why were you doing that work to figure out these ideal customer profiles? Ultimately, you know, the answer will boil down to something around improving the performance of the business.
So you might say, Oh, I want to do ICPs. Because I want to do better personalized marketing. Well, why do you want to do better personalized marketing? Well, I want to do better personalized marketing because I want to deliver more relevant information to my customers at the right time. But why do you want to do that?
And you keep asking why 4567 times? A lot of people say ask why five times and you get to the real root of the issue and ultimately boiled down to is you want to hire NPS net promoter score mean happier customers and you want to generate more [00:39:00] revenue and more profit. That's what every organization as well as nonprofits, not for profits, tax status, not a business model. All of you guys need to make margin on your products, and you do, but you need to think about it that way.
The bottom line is real simple that, you know, you were trying to improve the business. And so what you could ask the AI is to say, Hey, this is the background on my business. I'm trying to improve it.
Give me some ideas on improve it. Now, right now, every time you talk to chat GPT, you're starting basically from scratch. You know, it's like talking to kind of an idiot savant in a way where it's like someone who has all this world knowledge but forgets who you are like 30 seconds after you ask them the last question and that's a limitation that's going to go away very soon.
And in fact, in many respects, it has already because you can create custom GPTs, which we've talked about and incorporate a bunch of background information. In your custom GPT. So you could, for example, have like a detailed document about your business, put it into a custom GPT and that becomes your business expert and then go ask that custom GPT.
Hey, I'd like to increase my revenue. What are the things that I should do to focus on increasing revenue? [00:40:00] And it might give you some suggestions. Now this is where agents come in because you might say, Oh, like maybe one of the suggestions that came up with is improve your marketing personalization. Mallory, your marketing is very bland. It's very generic. And you say, that sounds really good. Mr. AI, okay, cool. Can you go do that for me? And the AI says, sure I can. Let me invoke the sidecar AI agent. And the sidecar going to say, okay, I'm going to go do this 15 step recipe to basically figure out the ICPs. It's going to pull some data, it's going to generate ICPs. It's going to like then test them against like industry benchmarks and whatever else. I'm just making stuff up right now.
So I'm hallucinating, I guess you could say.
But, essentially, you know, what you have there is. The ability to stitch these concepts together.
The tooling is there. You just have to be a little bit creative and how you use it. Yesterday I was working with a software engineer on a complex technical problem, and we were looking at the way a language model is being basically interacted with through some of this code, and we're looking at it saying, Okay, well, How do we ask the question?
And really, my input [00:41:00] to this engineer was, well, think about if you were asking me that question. Did you give me enough information to give you an answer? And then shortly after the answer was, well, no. And then the change the code actually was very simple. It's just if you were talking to a person, you could ask the question better and you get a different result.
So short version of my answer is 100%. You can do things today and going back to what you're saying about being proactive. I think our agents in the future will come to you actively and say, Hey, I know you always want to be looking to improve your business. You don't have to ask me for ideas to improve the business.
I'm going to come to you every week with suggestions based on everything you've done this week. Mallory, these are the things that I think you could do better next week, right? And automatically suggest those things and even offer to then do those things for you if you like the ideas. So there's a lot of opportunity around that and coming back to structure data, which we're talking about at the moment,
You know, not having to dig for those insights, being able to be given suggestions by the AI. This is not science fiction. This is stuff that, you know, as I said, you can build it now [00:42:00] with a little bit of ingenuity and a little bit of effort. and the things that we're gonna have very soon will automate this to a large extent.
The one other thing I wanted to mention, and this ties back to our earlier comment about the upcoming webinar again. That's on January 18th at 11 a. m. Central and at noon Eastern. It's information is available at sidecarglobal.com/data.
That webinar, we're gonna talk all about this. And so we're gonna talk about these problems and we're gonna talk about a solution and a solution that we're going to discuss is actually the same one we've used at Blue Cypress for some time.
So Blue Cypress is a family of over a dozen companies. These companies are brands that you probably are familiar with. These are companies that serve the association sector, and they all use HubSpot as their CRM, and they use a variety of other systems. And so we at Blue Cypress needed to be able to see the data across all these different structured sources.
And so we used an open source common data platform. And that allowed us to bring all of our data together into a unified simple environment, which we can then do analysis on. We can do [00:43:00] AI driven interaction with it. There's a lot we can do with it because we brought the data together from these different structured sources into a common data platform.
Again, we're going to be talking about that at length on January 18th.
Mallory Mejias: Is that an essential step? I mean, to have all of the data in one place or is AI able to access it even if it's siloed and in different platforms?
Amith Nagarajan: I mean, AI is capable of accessing data anywhere where you give it access. The challenge is that if you don't have it all in one place, it just makes the problem much harder, and it makes it also less flexible for you. Some of the types of AI. Do require you to have your data in one place. For example, if you want to train a model based on the data set using machine learning or You know, even feeding data to a language model, you need to be able to have easy access to it.
So I think solving the data location problem as a primary goal is a really important thing to do. It isn't that AI. Can't weave itself into all these different, you know, places extend its tentacles, so to speak, across your enterprise is [00:44:00] just a lot harder to architect a solution that way. And then you end up with a very brittle architecture, because if any of those bits and pieces change, the whole thing breaks, which you really want is a foundation.
You want a bedrock that you can build on top of, and that's why you don't, in my opinion, want to build your AI. With these tentacles and all these different systems, you want to solve for the data location and data unification first. And then build your AI strategy on top of that. And it's not as hard as it sounds.
There are ways to approach that that are well within reach of the association market.
Mallory Mejias: Final topic we are covering today is exhaust streams, and I'd actually not heard this term prior to you mentioning it, but it makes a ton of sense. Exhaust streams encapsulate the byproducts or the exhaust of online interactions and digital activities. This includes data generated from email, marketing, analytics, website, traffic patterns, social media, engagement, metrics, likes, shares, comments, and user behaviors on community platforms.
For associations and nonprofits, these exhaust streams are often [00:45:00] untapped reservoirs of insights, offering you a deeper understanding of member engagement, content effectiveness, and overall digital footprint. Analyzing these streams can reveal patterns and trends that aren't immediately apparent, guiding more informed decision making and strategy development.
Like with all other types of data, the challenge and opportunity lie in harnessing this data effectively. With AI, organizations can sift through these vast unstructured data sets and extract those insights that can drive strategies and your content personalization and your targeted outreach efforts. Amith, what are your thoughts on exhaust streams and how can associations and nonprofits leverage those?
Amith Nagarajan: Well, I think it's a really important concept to be aware of as a starting point. So people don't really think of this stuff. Most of the time, even folks that are like deep in systems thinking and are thinking about data a lot, they don't necessarily think of this. And it's an opportunity that's wasted to an extent if you don't have that information flowing through your head as it's just an [00:46:00] opportunity.
So let's talk about a couple things. Associations tend to want to know about their members. They want to know what people are interested in. They want to know personality styles, perhaps. They want to know about preferences of various kinds. And so the age old method of trying to figure that out is for me to send you a survey and say, Hey Mallory, can you take 10 minutes of your time and fill out this 30 question survey?
And I'm going to send it to you every year so you can keep us up to date. On your areas of interest perhaps some other traits that might be helpful for us in personalizing your experience sounds pretty good.
In theory, the problem is, is there's a low participation rate. So if I send it out to 1000 people, maybe I get 30 to 50 percent to come back. The others don't respond. And those that do respond. One of the things that's really interesting about surveys is people are actually really, really bad at predicting what they will be interested in. They're very good at telling you what they were interested in, not good at telling you what they will be interested in. And that's fair, because they don't necessarily [00:47:00] know.
A lot of times people assume, for example, that, oh, I attended a conference session on topic A. And topic A is, therefore, what Amith is really interested in. And so let's send him more content on that topic. And maybe that's the case. But a lot of times actually it kind of means that I've exhausted my interest in that topic because I attended a session on it.
Maybe I read six articles on it in the last six months. And so the prediction of where you want to go needs to be a little bit more real time. And so the problem with surveys in this context is that they tend to be a snapshot in time. They're poor predictors of where people want to go. And guess what?
You actually have a lot of information that the survey doesn't tell you, and it's from these exhaust streams. So, I'll give you an example. Going back to the online community platform. If you have people having conversations on your platforms, You have rich text data, and you can take that text data, run it through some really basic language modeling type of, you know, routines, and from there extract topical insights, [00:48:00] personality style insights, a lot of other preferential insights that you can gain just by looking at the content. So, That's a process that's totally achievable.
And you know, again, it's something that, would give you the ability to then have some structured data that is derived from the unstructured data of the community. So I'll give you an example. I post an article about topic B on your online community. My online survey from a year ago said I'm interested primarily in topic a But I actually really showed interest and maybe some level of expertise in topic B and I can tell if you're an expert in that topic or if you're a novice based on what you wrote.
And through that information, I can personalize my marketing. I can send an article to that individual that's about topic B. Or if I think, Oh, wow, that person clearly is well respected in my community on that topic and we don't have A lot of people with that expertise. And in fact, it would be great for us to have a speaker on that topic at our upcoming meeting. Let's invite that [00:49:00] individual to be a speaker or invite that person to submit a proposal to speak. There's all these really cool workflows that you can put in place through language models and through agents that take advantage of these exhaust streams.
I'll give you one more example that I think is like a real sleeper.
It's your emails. So email is the most frequent type of contact you have with people. Online communities are awesome, but unfortunately they typically only penetrate about 10%, maybe 20 percent of your population. Most people don't go to your online community in any predictable way. And improving that, of course, is a, is an honorable goal.
But, you know, it's, it's a challenge because it's a deeper form of engagement. But you do have emails that go to everyone and you probably have pretty good engagement rates with your emails, even in fact, knowing what people open and don't open that by itself is actually a form of data.
Because if I know you're more likely to open emails at a certain time of day or a certain day of the week, Or perhaps with certain types of subject lines that are positive or negative, or perhaps emails that have certain [00:50:00] keywords or certain topics or categories of topics. That's really interesting information for me to have on you.
And I have that information based on your open rates, relative to topics, relative to times of day of send. I have that data certainly on your click rates, too, because when I have your clicks, I know the articles that you clicked on in your newsletter or the offers that you clicked on in e commerce style emails.
Rasa dot io, which is one of our companies. They trade in this data, meaning that they provide tons of assistance to their clients based on this exhaust stream of topical insight and personality style insight that they can sink right back to your AMS or CRM and that's actually one of the greatest value adds to that product.
It's not so much that the newsletter is great and people love it. That's that's true as well. But the exhaust stream from that product is extremely powerful.
Mallory Mejias: I want to dig into these exhaust streams even more with sidecar as an example. So we have a community, right? We send emails, we send a newsletter. So let's say we have someone on our community that is engaging with a lot of the AI [00:51:00] content that we post. And then they're also, when we send emails about AI events, we have, they're clicking on those emails, they're viewing event AI landing pages on our website, they're clicking AI articles in the newsletter, right? We can understand that their topic A, , in your example, at least at this point, is AI. They're interested in AI and we think they might be a good fit for something like our AI boot camp. Is the CDP, the common data platform, a place where you can consolidate all of that, those exhaust streams or are there, do you have to make integrations for that? How does that work?
Amith Nagarajan: That's exactly the idea Mallory with the CDP is that if you have a CDP, which is again a common data platform, basically a large data repository where you can pull in all of these different disparate data sources. Your exhaust streams can tuck in really nicely in there. So, for example, that particular attribute of data could go right into the sidecar CDP.
And then from there, you could use that for marketing. You could use that for content personalization. You could use that for all sorts of cool things. So the CDP is a great place to store that. [00:52:00] Now, from the CDP, you might want to push that data back into other systems. It may be useful to have that in HubSpot.
It may be useful to have that in your AMS. For associations that use an AMS, you know, there's lots of places you might want to push the data. But as a primary goal, getting into your CDP. Is step number one. And then from there you can distribute that data out to wherever it needs to go if you'd like to.
Mallory Mejias: Okay. So first step is consolidating that data all into one place and then perhaps pushing it out to your CRM or maybe your AMS, if that's what you use.
Amith Nagarajan: Essentially. And you know, and those steps depend upon the type of data. So certain that what you're describing, I think would be super valuable to have in an AMS. But you know, necessarily in everyone's case, some people use an AMS strictly for basic membership processing, and they don't really need that level of granularity.
But other people use it as more of their central hub. And so having it in AMS is a really good idea. The idea of a CDP, though, is distinctly different from what an AMS would do. It's essentially a read only repository of [00:53:00] data that essentially represents the amalgamation of all the other data that you have across the enterprise in one place.
And so the power behind that is then you can unify that data, make sense of it together, and then you can build your AI strategy on top of that. Of course, you can also do analytics. You can do a whole bunch of other cool stuff. You can drive a much better web experience to from that unified. Data source compared to the kind of disjointed web experience, most associations unfortunately have. You can solve a lot of problems with the CDP. But the primary thing we've been talking about is having that data in a unified location so that you can in turn run appropriate AI on top of it. But it does provide a good integration strategy generally because you know what most people have is an integration strategy.
Looks kind of like a bowl of spaghetti, you know, where there's just all these lines drawn all over the place and you really can't make heads or tails out of them in terms of which line connects which system to which other system. All that creates brittleness and it's easy for it to break. It also creates way more vendor lock in.
Because if my AMS. Has 15 distinct [00:54:00] integrations with 15 different systems, It's so hard to maintain those and to even consider changing systems is very, very difficult. Whereas if you have the AMS integrating with one thing, which is a CDP and all of your other systems integrate with the CDP, it creates a really clean environment. It's more of a hub and spoke model, but the key is the CDP something you own.
Mallory Mejias: That makes sense. I feel like with exhaust streams, it would be easy to just uncover millions of data points, probably even more if you're looking at things like length of time on a page or a click or a like or a comment, so on and so forth. Do you think it's best practice to have a human or maybe an AI beforehand establish which exhaust stream data types are most important, or do you think it's best case to collect absolutely everything that you can and then leverage it later?
Amith Nagarajan: I think it's good to establish some thought process around what might be valuable and what might not be, but not to think through it too hard. It's kind of like coming up with a pricing strategy for a product. [00:55:00] You can do a lot of theoretical work, you can do some market research, but just getting out there and selling your product is one of the best ways you can find out if your pricing is any good.
Similarly with these things, until you bring them in, you're probably not going to have a really good idea of how the exhaust streams could be used. But once they're there, they might represent themselves to you in a way that you're like, Oh, that could be really interesting. Once again, AI can be helpful because if the AI is aware of the complex, and comprehensive nature of your enterprise data catalog, basically, which is what this is.
The AI can make suggestions. The AI can say, Well, actually, for your marketing, you might want to consider using this piece of data. So there's a lot of really cool things where we talked a lot in this podcast about human plus AI being A really interesting of ideas, and this is a great way you can leverage AI S. To give you ideas and how you can use that data as well.
Exhaust streams are super interesting things to think about. I'll give you one more example. A lot of people probably haven't thought of, which is your events. So you have these videos and when you're doing video recording, most people record the speaker.
[00:56:00] Well, it's possible to also record the audience. There are some privacy concerns there, but if you get consent and you let people know you're going to be recording the room, and you also, I think, from my perspective, importantly, do not use facial recognition. So you're not trying to figure out, like, the facial reactions of each individual at the, at the identifiable level, but you look at it in terms of the crowd.
How full is the room? How empty is the room? How many people came into the room? During the talk, how many people left the room during the talk? How many people were engaged in paying attention to the speaker during the talk? That's really interesting, valuable data if you think about it.
Once again, how do we find out if a speaker was good?
Right now, we run a survey. We ask people to fill out a survey to tell you what they think of the speaker after the event. That's okay. But, you know, it's, it's tough because that's also an area where is it truly objective or am I being kind of nice because most people give bad grades to folks sometimes they don't mind, but a lot of times it's, you know, skewed in one direction, the other,
And it really takes an awful or a really great speaker to really get people to fill those things [00:57:00] out in my experience. So instead, if you look at the audience and just kind of gauge, people are pretty good storybooks. you know, if you look at someone's face while they're listening to something you can tell where they engaged, where they not? Were they on the phone the whole time or on their laptop? Were they, you know, actually doing something? Are they processing the information, so to speak?
So that's one exhaust stream that I think is super simple, and would be very easy to run a video analysis like that and then rate the session.
You wouldn't, it wouldn't take a lot of work to do something like that. You could even run a video clip through a basic AI to ask for the overall level of audience engagement on a scale of 1 to 10.
There's a lot of cool stuff you can do with that. So really powerful exhaust streams are everywhere. And, you know, as you start thinking about this actively like, Oh, what's my business process? What's the byproduct of that business process? Oh, I'm constantly doing member service. The byproduct of it is I have lots of emails back and forth between my members and my team.
And guess what? The best performing member services representatives in terms of customer satisfaction That's interesting training data because the questions And the responses that [00:58:00] particular rep gave, you might want to clone.
And then also it's instructive to look at the worst performing reps and say, well, that's what we don't want to do.
And guess what? That exhaust stream is super useful for training models. So there's a lot of things like this out there. I get really excited about it because I think the landscape is far, far richer.
When you think about data the way we're talking about it, you go from a black and white image to all of a sudden full vivid color and you realize how much depth and how much, you know, detail there is in the data landscape of your association literally passing by you every day.
Mallory Mejias: I feel like we could talk about this forever. I have more questions, but if you enjoyed today's episode and you want to get more of this content, highly encourage you to register for our webinar January 18th at 11 a.m. Central Noon Eastern. It's called Own Your Data, Own Your Future. Amith is leading that webinar and you can register for it at sidecarglobal.com/data. And on that note, if you're looking for more AI education in 2024, check out our AI Learning Hub. We [00:59:00] have flexible on demand lessons. We have weekly office hours with AI. experts, and you get access to a growing community of fellow AI. enthusiasts. Amith, thanks so much. I'll see you next week.
Amith Nagarajan: Thanks for tuning into Sidecar Sync this week. Looking to dive deeper? Download your free copy of our new book, Ascend, Unlocking the Power of AI for Associations at ascendbook. org. It's packed with insights to power your association's journey with AI. And remember, Sidecar is here with more resources for webinars to boot camps to help you stay ahead in the association world.
We'll catch you in the next episode. Until then, keep learning, keep growing, and keep disrupting.

January 11, 2024