Show Notes
In Episode 8, Amith and Mallory decode recent research findings in the field of AI interpretability, shedding light on how and why AI models make certain decisions. They also explore Mistral AI's Mixtral 8x7B model, which utilizes a 'sparse mixture of experts' (MoE) architecture for enhanced efficiency and effectiveness. They round off the episode on the EU's new AI Act, discussing its implications for global AI governance and U.S.-based associations.
Let us know what you think about the podcast. Drop your questions or comments in the Sidecar community: https://community.sidecarglobal.com/c/sidecar-sync/
Join the AI Learning Hub for Associations: https://sidecarglobal.com/bootcamp
Download Ascend: Unlocking the Power of AI for Associations: https://sidecarglobal.com/AI
Join the CEO AI Mastermind Group: https://sidecarglobal.com/association-ceo-mastermind-2024/
Thanks to this episode’s sponsors!
- AI Learning Hub for Associations: https://sidecarglobal.com/bootcamp
Tools/Experiments mentioned:
- Betty Bot: https://bettybot.ai/
- ChatGPT: https://chat.openai.com/
- Claude 2: https://claude.ai
- Google Gemini: https://deepmind.google/technologies/gemini/#introduction
- Mistral AI: https://mistral.ai/news/mixtral-of-experts/
Topics/Resources Mentioned:
- AI Interpretability: (Last segment of episode) https://podcasts.apple.com/us/podcast/2024-big-ideas-miracle-drugs-programmable-medicine/id842818711?i=1000637961888
- Mistral Unveils Mixtral 8x7B: A Leading Open SMoE Model: https://www.maginative.com/article/mistral-unveils-mixtral-8x7b-a-leading-open-smoe-model/
- Turn the Ship Around by David Marquet: https://a.co/d/4IcDnTl
- Artificial intelligence act: Council and Parliament strike a deal on the first rules for AI in the world: https://www.consilium.europa.eu/en/press/press-releases/2023/12/09/artificial-intelligence-act-council-and-parliament-strike-a-deal-on-the-first-worldwide-rules-for-ai/#:~:text=The%20draft%20regulation%20aims%20to,huge%20milestone%20towards%20the%20future!ChatGPT
Social:
- Follow Sidecar on LinkedIn: https://www.linkedin.com/company/sidecar-global
- Amith: https://www.linkedin.com/in/amithnagarajan/
- Mallory: https://www.linkedin.com/in/mallorymejias/
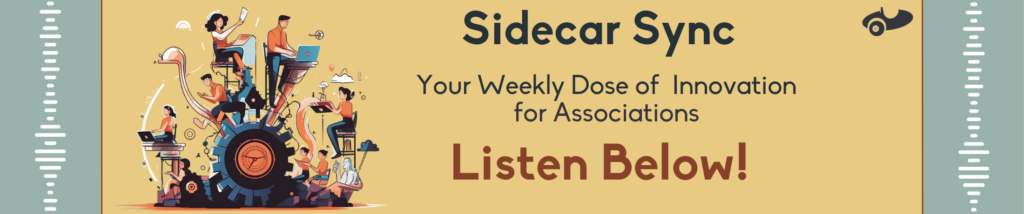
Amith Nagarajan: Hello everybody. And welcome to the latest episode of Sidecar Sync. We're [00:01:00] super excited to be with you and appreciate you spending some time with us to learn about the latest and emerging technologies like artificial intelligence and how they apply to your association.
Before we get going, I'd like to take a moment to thank our sponsor. This week, Sidecar's very own AI Learning Hub. The AI Learning Hub Sidecar Offers is designed to help you both start and continue your learning journey with AI throughout the year. You gain 12 months of access to over 30 lessons, detailing advanced use cases of AI, as well as very basic beginner entry level things.
The AI Learning Hub is helpful for you and everyone on your staff and Sidecar offers options for individual registration, as well as association wide, all staff access, learn more at sidecarglobal.com/bootcamp.
Mallory Mejias: Hello, everyone, you've probably heard us talk quite a bit about the digitalNow conference here on this podcast, and we've got some really exciting news, exclusive for Sidecar Sync listeners only, you're the first group of people to [00:02:00] hear this information, but we have locked in the dates and location for digitalNow 2024. Those dates will be Sunday, October 27th to Wednesday, October 30th in 2024. And the location will be Washington D.C. Amith, are you excited about that?
Amith Nagarajan: I sure am. digitalNow has never been in DC in its 20 plus year history, and we're excited to bring the event to our nation's capital and the capital of the association industry in terms of being the location that has the largest number of associations anywhere on planet earth as far as I'm aware.
Mallory Mejias: That is true. So everyone be on the lookout for that official announcement and we plan to run a special offer for those first few people to register. So be on the lookout for that. I'm excited to kick off today's episode with topic number one, which is AI interpretability. So we've mentioned this a little bit on the podcast before, but we haven't really explored it in depth, so I'm looking forward to doing that today. This [00:03:00] topic sits at the heart of understanding artificial intelligence by addressing critical questions like, “why do AI models make specific decisions and what drives the varied performance of different models.” As AI continues to integrate into various aspects of our lives, unraveling this black box of these systems becomes increasingly essential.
So large language models, or LLMs, are composed of numerous neurons. And you can think of these neurons as being a single computational unit within the model. Traditionally, efforts to understand AI focused on individual neurons, trying to decipher their specific roles and functions. However, this approach provides a limited view of the model, and the challenge lies in deciphering the rationale behind a model's behavior when you're looking at a specific neuron. The field is now evolving towards a more comprehensive understanding of AI models with the focus shifting to something called features. Features represent a concept that activates a particular set of [00:04:00] neurons for specific reasons. Unlike individual neurons that might be involved in various tasks, a feature could activate a cluster of neurons for a specific topic like climate change, for example, providing a clearer insight into how the model processes information related to a particular subject or output.
This transition from a granular focus on individual neurons to a broader understanding of features represents a significant advancement in AI interpretability. It's a shift from looking at isolated components to understanding how groups of these components work together to create meaningful outputs.
Understanding more about how these models work means we can have more control over them and ultimately, more control means more reliability, which is especially important when you're using an AI model for something that is mission. Amith, why should associations care about AI interpretability and what is it?
[00:05:00]
Amith Nagarajan: Well, 2023, I think will go down as a significant year in AI for a lot of reasons but one of the reasons I think that is often under the radar is this topic of interpretability. And when people talking about safety and AI ethics and a variety of topics that kind of are under this umbrella of can you have safe use of these models?
Interpretability often isn't really discussed, and it should be because interpretability basically is telling you why the model acts a certain way. So in traditional computer programs, they're built in a way where there's essentially a bunch of rules coded in by people into the program and the program does a certain set of things.
And so you know exactly why it behaves a certain way. Why does your financial system process an invoice a certain way? Well, because those rules were codified into the program. In comparison though, the way AI models work is not deterministic. Meaning that you don't exactly know what the output is going to be for the same input.
You can test this yourself. If you go to chat GPT, for example, or Anthropic’s Claude and put in the same prompt multiple different [00:06:00] times in different conversations, you will get different answers. And it's a similar thing to again, if you might speak to a person and ask a question, even the same person at different point in time, you get a slightly different answer. And so interpretability aims to essentially lift the veil on the mystery of how these models work. As you've described well, the neurons in the models are individual computing elements, but they're tiny and they're like in a biological brain. There are many of them, so billions and billions of them, even trillions of them.
The human brain is estimated to have 100 trillion neurons as an example or point of reference, but you know, these largest models are just crossing the one trillion range of neurons. The point is, is that at that scale, they do such a small, tiny thing that it's hard to really understand what the impact is.
So, what researchers have been shifting towards is this idea of features, which is groups of functionality. It's more of a logical thing you'd think about, like the example you provided around a topic like climate [00:07:00] change or a particular skill. For example, being able to create a blog post might activate a feature or a group of neurons that are different than something like interpreting a blog post.
So, it might be a different part of essentially of the artificial intelligence model. Coming back to your question about why associations should care. I give you two answers. So the first one is everyone should care because models should not be a mystery. We should understand how these things work in much more resolution so that we can then have more predictability and that leads to safety.
You know, it's kind of like this. Imagine if once upon a time back when cars were first introduced to the roads and, you know, he had a car and it was great and it worked and it worked and then all of a sudden the car took off and started flying. It's unexpected. In the AI world, you would call that an emergent property or an emergent behavior, which is something you didn't necessarily expect the model to be able to do, but the model all of a sudden started doing it and you'd go, [00:08:00] wow, that's really cool that my car can fly.
But you might be curious how that's possible. You might be curious why it's doing that, and you'd be right to be not only curious, but perhaps concerned, especially if you were in the vehicle at the time. And so I would extend that analogy back to AI to say that many of these models do things that we don't really understand. We didn't expect them to be able to work at this level, but as the scaling laws have continued to show, we have these emergent properties coming out of models that are truly stunning, but we need to understand better how they work and why they work a certain way in order to get to improved reliability.
Which in turn also leads us down a path of dramatically improved safety, so it's important for everyone. And then finally for associations, what I would say specifically is you are going to want to put AI out there in front of your members, in front of your audiences. And you're going to want to know what these things are doing.
You know, you can put products out there that allow you to interact with your members and audience through your content and through AI. And these early [00:09:00] models work extremely well, but it would be great for you to have a much greater visibility into, you know, why they work a certain way as you're representing, you know, accurate answers to your audiences.
So I think it's important for everyone in associations critically need to make sure they're right about the information they provide.
Mallory Mejias: Digging into this idea of emergent behaviors, I think that's a really interesting example you gave with the flying cars. Is it safe to say that even the creators of these AI models don't fully understand what they've created?
Amith Nagarajan: Yes, that's 100 percent correct. Every researcher out there who talks about this stuff that I've ever heard speak or write always says the same thing because none of them are professing to have, you know, some kind of inside scoop. These models essentially have been black boxes. And as amazing as their capabilities are, that's actually one of the main reasons safety advocates have been so concerned because the lack of interpretability historically means essentially that these, you know, what these models are gonna do next is, you know, not really clear.
It's very, very unclear to people. Now, let's keep in mind [00:10:00] something about reliability and safety. Going back to the flying and the driving you know, analogy. So if I were to tell you that there's this new invention called an airplane and you're amazed by it, you think it's wonderful, it can, you know, bring people closer together, transport goods and services, all these other wonderful things an airplane can do, but you're worried because, you know, taking to the skies means there might be some crashes, there may be death, there may be all these negative problems with flying, there's risks of it.
Well, of course, you'd want to reduce that risk to as low as you possibly could. But would it mean that you wouldn't tolerate it flying as a modality of transportation if the risk weren't zero and the answer is clearly not there's an acceptable level of risk. There's a reasonable level of risk for everything in life.
And so with flying, you know We know that it's incredibly safe to fly far far far safer than driving a car on the interstate, for example, here in the U. S. but it still has some risk. And similarly with AI interpret ability isn't going to get us to zero risk, but it's gonna dramatically reduced risk because we're gonna understand [00:11:00] how these models work much, much better.
That will also lead to better models in the future, because we'll be able to understand how to train them better on many other downstream effects. So it's gonna be really exciting to see what happens. And there's a lot of momentum in the interpret ability field within AI. So we'll see even more innovation happen next year.
Mallory Mejias: So you mentioned that one feature might control how to write a blog and maybe another feature would be related to a topic, how do these features work for the everyday person?
Amith Nagarajan: Well, for an everyday person, you'd never really pay attention to it in a sense in that as you're using these models, you wouldn't be concerned about which feature or sub feature of a model is being activated. To use the product. Similarly, like when you make a phone call on your cell phone, you're not necessarily thinking about all the different pieces and parts of what's happening to make the magic of a cell phone call actually work.
Or when you get your, you know, the latest product you bought from, you know, whoever it is you bought a product from. How did that product get to you? So I think on the one hand, it's [00:12:00] kind of irrelevant to the end consumer in a way. But the flip side of it is, is that that under that interpret ability or the understanding of the inner workings of the model will allow people to first of all, design better systems if they understand the model better you'll understand the capabilities of the model. If you know that you have 5000 features that relate to blog post writing and only 200 features related to, let's say something like social media expertise in my marketing model, my marketing AI bot.
Then clearly that model is better at blog post writing, but of course that relates to probably how I train that model. And part of that again comes back to predictability and thinking about features versus neurons. It's just a little bit higher level concept. Neurons are so low level and they're layered in a way where they're really not understandable by anyone outside of, you know, people deep in the field.
But features are more abstract concept. So it's just a way of saying, hey, I can make interpret ability somewhat approachable to perhaps not the average end user, but [00:13:00] to at least people who are putting these systems together.
Mallory Mejias: We spend a lot of time talking on this podcast and certainly at Sidecar about practical applications of AI, which is important to understand what tools are out there and what they are capable of. How important is it, though, to understand? How these models work features, for example, neurons, for example, neural networks, what is the balance there of knowing how to practically apply AI tools and then actually understanding how they work?
Amith Nagarajan: So what I always hammer home with everyone I talk to in this space is that the first step is learning, which is part of what you're investing in doing and listening to this podcast or reading books or reading blog posts, et cetera. And so understanding that interpretability, first of all, exists. And secondly, is growing really fast so that it's going to improve is important because if you're in a room and you're discussing AI and you're discussing how your association may adopt AI and someone raises the question of, hey, from a safety perspective, we don't really understand what the model is [00:14:00] doing, so we can't deploy AI, which is a reasonable concern for someone to have.
If you're armed with the knowledge that interpretability is improving at a rapid pace, you can say, well, I definitely hear you on that concern. But let's talk about where interpret ability is and where it's likely to go over the next 12 months. Remember that AI is on a six month doubling cycle, meaning that it's doubling in its capability and performance or having in cost relative to performance every six months, which is an insane growth rate.
That's why this field is so hard to keep up with. And interpretability is going to grow at that same scale. Every major AI lab is focused on this, as are most universities deep in AI. The reason is, is that without interpretability, truly enterprise scale adoption isn't going to be possible. You're not going to have an insurance company make underwriting decisions purely through the AI model unless they have a high degree of interpretability.
That particular example isn't a great one because fintech and financial services companies are really advanced in this area and they have highly [00:15:00] specialized ML for years. They do exactly what I'm describing, but the point is, is that you're not gonna really hand over the reins to the AI fully for certain processes until you understand them better.
Again, think about people when you're thinking about drawing an analogy. If I just hired an employee and I never knew, I know nothing about them. I just know they're really good at a particular type of task. I wouldn't necessarily hand over everything to them until I understood how they work and I want to see their outputs.
But I might want to also talk to them and say, hey, tell me how you thought about this Mallory. You know, why did you make this decision on your, on your, on this particular piece of content? Or why did you write the marketing campaign that way? Being able to ask questions of the model essentially. And so I think that's a really important thing for people to be aware of first of all, because even though you're not going to think about interpretability every day necessarily. Knowing that it's there and it's something we can start to lean on more is really important in how you think about planning your future and your AI adoption. And again, I keep pointing out the six month doubling because interpret ability is really, really rudimentary right now.[00:16:00]
What I expect is that every major model will have an interpret ability panel or some kind of feature in their user interface where you'll be able to look at every interaction you have with AI. And you'll be able to see a breakdown of the parts of the model that were invoked in order to serve your request.
It might not be generated every single time because there is cost involved in, in executing these interpretability, you know, paths. But it's something that could be done, and that could be a major advancement for everyone's comfort level in understanding how these models work and their predictability.
Mallory Mejias: So is the idea that we would see the features, the clusters of neurons that are activated for a certain task or a certain topic, and then if the model performed in a way that didn't align with what we were looking for, we could then tap into that feature, make an edit there, and then kind of solve the problem?
Amith Nagarajan: Yeah, the term alignment you're using is fantastic because that's exactly what people in the industry are starting to refer to the broader safety conversation is how do you align the model with your overarching objectives? How do [00:17:00] you align it for specific tasks? And so if I know that certain features are being turned on or turned off based upon the nature of a request I have or the way I trained it, I can change my training approach and I can also change the way I prompt the model in order to actually ask the model to use or to not use certain features. Like right now, mdels are not feature aware, so to speak, but these models will become increasingly sophisticated and able to understand, you know, their own capabilities more through this interpretability. And so if you want to essentially tune a model or prompt a model in a very, very specialized way in order to ultimately result in certain features not being used, right, then that's going to be much, much easier as a way of using the model.
It's also going to be great for people who are doing so called red teaming in the safety work for every major public facing model. Companies have a responsibility to do essentially like hacking the model, trying to break it to make it do things that are inappropriate or share information that the model companies in their various [00:18:00] levels of comfort are willing or not willing to let the models do.
This will make that much, much more straightforward because you have a higher level order rather than like giving the model feedback on every single possible scenario of like oh, don't answer questions about biological weapons. Don't answer questions about this or that. You'll be able to actually look at entire features or categories of features and deactivate them.
So I think that's a pretty important concept. This is all still pretty early in the research, but what's been proven so far with the work that we're discussing right now, there were some papers that came out this fall that showed definitively this mechanistic interpretability idea along with other forms of interpretability that are advancing as well is a, is a working way of actually identifying features and very clearly being able to have greater control through that.
What's happened so far is it's worked on small models. So researchers have basically done this on tiny, tiny models that you can run locally and they've experimented with. Now it's an engineering problem. Now it's a problem to say, how do you scale this up to [00:19:00] something of the order of GPT 3.5 or even GPT 4 and beyond that where we're heading.
So there will be a bit of a game essentially played between the researchers who are trying to have interpretability, keep up with the power of the models that are coming out. And of course the models themselves, which are not slowing down, but I'm very optimistic. I think 2024, we'll start to see all the mainstream language models and other models.
Start to talk about interpret ability publicly and have feature sets that relate to this. I'm quite confident you're gonna see that because it's going to have a big impact on enterprise adoption.
Mallory Mejias: So do you see associations being able to fine tune these feature sets, for example, or do you think we'll be relying on the AI giants in 2024 to make those changes?
Amith Nagarajan: That's a great question. I think that with the open-source world, there will probably be a lot of control that you'll have. I don't know if it'll be through fine tuning or lead through some other method of configuring the models after they're fully trained. But I think you'll see in the open-source models a lot more control, as is natural in that universe.
And [00:20:00] I do think the vendors like Anthropic and OpenAI and others are going to provide more and more and more granular control over these models, particularly for their enterprise customers. I think associations should plan on having access to some level of interpretability functionality next year.
Now, depending on the association and where they're at, if they're at the very beginning of the journey with AI, probably doesn't matter so much, at least for the first half of 2024 again. It's just a really important point. A lot of the stuff we talk about Mallory is more about loading up these ideas in your brain so that you have some idea about what this topic is.
And that way, when you're thinking about how to do things for your own role in your business, for your association, for your market, or even in your personal life, you have it in your back pocket, so to speak, and then you can go dig deeper and learn more about it if you need to. But I think interoperability is exactly one of those things that may not have kind of the sizzle effect of like, oh, there's a vision model or GPT 5 or 5 is reportedly going to be released before the year's over and all that kind of stuff.
Those are all [00:21:00] sizzle factor. But interpretability, I really think is the thing that's going to drive much broader enterprise adoption for the current technology we have, which is, we all know, is already extremely powerful.
Mallory Mejias: I completely agree. When I first really started to understand the fact that Chat GPT, for example, was, was just a next word predictor and really understanding what that means. I feel like it unlocked a whole new world of AI for me and understanding what hallucinations were and how that worked. So I think interpretability, you're right, is that same thing.
Maybe it doesn't have the sizzle factor, but it helps you kind of understand what these models are capable of and how they might change in the future.
Amith Nagarajan: You know, and you bring up hallucinations are a great related topic for interpretability because, you know, some people might say that their initial reaction is, oh, I don't want that to happen at all. But in fact, actually hallucinations, of course, they can be a bug. But hallucinations actually can also be a feature and let's just talk about you and me, for example, along with our other 8 billion friends on the planet, we run on software in our brains and we hallucinate [00:22:00] all the time.
Every time we come up with a novel idea, it's a hallucination. We don't necessarily have a pattern of prior data that suggests that we should be thinking about launching the Sidecar Sync podcast necessarily, or, you know, doing something else. There may be certain clues, but each original work or each novel idea yes, of course, it comes from some sense of prior patterns, but there's a lot of hallucination going on that drives creativity. Certainly that's the case for artists. There's actual hallucinations going on, but, you know, we've got a lot of that going on in our own minds, which is a feature and a bug.
And, you know, the question is, is are you hallucinating or not? Do you want a system to hallucinate if it's answering factual questions about medical conditions? The answer is probably not, right? Or definitively not. Whereas if you have a tool that's like, hey, our association is gonna put a tool out there for our members and it's gonna be a brainstorming partner.
It's gonna help those organizations that are using this tool to come up with new novel products and ideas for their industry, right? And I'm talking to associations [00:23:00] right now who are thinking about building stuff like this several of them and it's exciting because they have all this great knowledge and in that application, well, you probably want to be a little more freewheeling.
You probably want to say actually AI go ahead and hallucinate a little bit. Let's see what you come up with. I mean, Dolly 3 or Mid Journey or Stable Diffusion, those image models, they are hallucinations, the whole thing, right? And even many of the things that you get out of language models are forms of hallucination.
The problem is, is the model doesn't know when it's hallucinating. Like, if you talk to someone who's basically really full of it, but they start believing their own press and they think they're, they're actually quoting you facts. That's when it gets really scary. I mean, it's scary in general, but like when, you know, when you run into people like that that is really, really scary.
And that's the problem with these models is they're so incredibly good at convincing you with this definitive language that they know exactly what they're talking about. And reality is, is that they don't know if they hallucinated or not. And that's a big part of interpretability is to be able to say definitively, this is based on fact, this is not how much of the creative feature set, [00:24:00] so to speak, of the model was used.
And that's where we're going with this stuff. So we're basically getting better insight into all of those aspects.
Mallory Mejias: Interesting. So hallucinations themselves, not so bad. Hallucinating and not knowing you're hallucinating could be bad.
Amith Nagarajan: I would, I would say it exactly that way. That's, that's well put.
Mallory Mejias: Well, Amith, you, you mentioned open source just now, and that kind of sets us up to go into our next topic of the day, which is all about Mistral AI, which we've talked about before in the podcast and this mixture of experts architecture in its new model. So we're talking about a new development from Mistral AI, and it's a new model called Mixtral 8x7B.
What makes Mixtral 8x7B stand out is its use of sparse mixture of experts architecture. That might sound complex, but it's essentially a way for the AI to smartly choose the best tools from its kit for each specific task it's given. The model utilizes multiple specialized sub models or experts to handle different aspects of a [00:25:00] task.
This approach allows Mixtral to be both powerful and cost effective as it only uses the parts of its system that are needed for each job. It's said to match or exceed the performance of models like Llama and GPT 3.5 in evaluations, and it's particularly good at language generation over long contexts-32,000 tokens, code generation, and achieving top instruction following scores among open models. Mistral also reports the model displays higher truthfulness and less bias on benchmarks compared to other models like Llama2. Mistral AI's decision to make Mixtral's technology open to the public is also significant.
It means that developers and organizations, including associations, can use this model to innovate and create new applications that could benefit their communities. All right, Amith, can you explain the significance of this sparse mixture of experts architecture and how it differs from traditional AI models?
Amith Nagarajan: Sure, I'll try to provide a really high level of that. But before I jump into [00:26:00] that, let me just recap for some of our listeners who may not be as familiar with what's available in the open-source world. There are tons and tons of open-source models out there. So, things that are essentially comparable to GPT 3.5 and certainly GPT 3. There are open-source models for images. They're open-source models for video. There's open source all over the place. The reason that's an important thing to not only be aware of, but possibly to experiment with is you have a lot of choice and choice leads to competition.
That leads, of course, to lower pricing, lower cost, but it also leads to innovation. And that's part of what makes a I so exciting right now. It's like the early days of the automotive industry where there are hundreds of car companies, everyone's coming up with something new and that's leading to tremendous innovation, rapid pace.
It's obviously a super immature industry, and as the industry matures, it'll probably, you know, consolidate to some extent. But in any event, open-source is really important because what essentially these companies are choosing to do is to share what previously would have been locked up in a vault, the source [00:27:00] code, and in this case, also the weights, which are basically the neurons that we were talking about earlier.
Sharing that for free with the general public to do whatever they want with in any in any product. And so, that's a really exciting thing because of two factors. One is what I already mentioned- cost. And the other part is, of course, the fact that you can then actually dissect these things and understand them better.
So a particularly cool thing about the model of experts or mixture of experts, I should say, is you have essentially a number of models packaged together into a single model. So think about it this way, you might have a world class piano player, and that person produces an amazing, you know, amazing music.
It's wonderful to listen to. You love it. And sometimes you just want to listen to the piano. And if all you want is piano music, that individual and that instrument are perfect. But sometimes you want something a little bit different. And so maybe you want an entire orchestra. And so that orchestra has a bunch of specialists in it.
You have violin players, you have every other instrument you can think of. And [00:28:00] while many musicians are capable of playing multiple instruments the piano player might be okay at other instruments, but they're absolutely incredible at piano. And so similarly models, think of them, once again, in that human analog, where we have models that have been trained in certain ways to be really good at particular things.
Perhaps, like we said earlier, writing blog posts. Posts or composing music or generating artwork. And so what mixed role is simply doing is saying, hey, we're gonna take a bunch of models and package them together. And each of these individual models are specialized in particular areas. And then in combination, these models can work as a team.
And therefore, the overall model, the mixed role model has eight different submodels, essentially, that can work together to produce higher quality output. So that's the basic idea. In fact, many of you are already using a mixture of experts model because GPT 4 is based on this approach. Nobody knows the exact number outside of open a I have how many different models are part of GPT four, but it's rumored to be [00:29:00] something in the order of 16 or 32 or some number along those lines.
And I think that trend is going to continue over time.
Mallory Mejias: This idea of different models or experts, smaller models or experts working together. To me sounds a little bit like AI interpretability. And maybe that's just because I've primed myself to be thinking of that based on our first topic. I know that they're different, but how exactly are they different?
Amith Nagarajan: Well, I mean, interpret ability I think will certainly be enhanced by the idea of MOE or mixture of experts because the different models each are smaller and the bigger model is the harder it is to interpret because of just the scaling of the size of it, the number of neurons and so forth. And so theoretically, if you have a bunch of smaller models, you might gain better insight through current state of the art interpretability techniques like what we were talking about in the earlier segment. But the other part of it, too, is just by having a separation of concern, so to speak, these models at the moment can have them have the sub models essentially team up to [00:30:00] answer a question.
Right now the models are not necessarily doing a lot of cross checking where they're checking each other's work. But that's certainly something that these models are going to be doing over time. There's some element of that right now in the way there they're routing architecture works. But essentially, it's just routing the request to different model or model sub models.
So, here's the way I would really recommend thinking about it. You know, to your point, yes, interpret ability is actually somewhat implicitly tied here because it can give you better visibility to the sub models that are actually being invoked. But the most important thing about mixture of experts is it's basically like having a team of people going after a problem rather than individual.
You know, one of my favorite business authors is a This guy named David Marquet, and he wrote a book a number of years ago called Turn the Ship Around. This guy was the captain of a nuclear submarine, the USS Santa Fe, and he inherited the ship when he was first promoted to captain. That's what he wanted to do his whole life, and he finally got promoted to captain of the USS [00:31:00] Santa Fe.
And when he inherited that boat, it was the worst performing sub in the entire fleet. He had 135 sailors, and within a year, he turned that same sub into the best performing sub in the entire fleet. And so his book is basically all about how he did that. It's a fascinating read, by the way. It's called Turn the Ship Around by David Marquet.
Great guy and really interesting story. Um, The reason I bring that up What he keeps repeating and reinforcing is that no matter how smart the other captains were of the other boats, he built, no matter how smart they were, he would always win in simulated combat or in real life situations. Because he figured out how to activate the brains of 135 sailors to actively participate in decision making rather than having them simply follow like, you know, follow rules from up high and basically be complete, you know, command and control.
He figured out a way to empower these folks so that they could learn and they could act in [00:32:00] their specialty area in a tremendously powerful way, which of course made his team much more excited to do their jobs. And it allowed him to have 135 active brains. So in a way, what he did is he had 135 sub models in his mixture of experts and running that ship.
So I just think of it as teamwork. It's AI working as a team and I think it's the future of where these things are going to go. We're going to get a lot of research going in terms of like, how many sub models is optimal? What kinds of models do you package together? For the big general purpose models like GPT4, you're going to see a lot of that because these things have to be able to tackle everything from poetry to writing technical documents so there's gonna be a lot of different submodels. But for your association think about it this way, you might have a submodel trained on, for example, some of your technical content. You might have another submodel trained on some of your policies, and these models might be experts in different parts of what you do and brought together into an overall MOE style of model that that serves your overall needs, and [00:33:00] that could be a very interesting development for associations to watch. For most associations, this is not something you're gonna go play with. Open-source in general probably is out of reach for most associations with their typical technical capabilities right now.
But like everything else we're talking about, it's moving fast, so this will impact you at some point. Once again, it's one of these things to be aware of and just having that in your knowledge that that's how these models are advancing is really, really exciting.
Mallory Mejias: So you can think of submodels as the sailors on the ship, like you mentioned, and then maybe the features conversation is more like which sailors came together to perform certain tasks. I know that's kind of a really basic analogy, but does that make sense?
Amith Nagarajan: Yeah, no, for sure. You can definitely think of it that way. Features are essentially grouping, you know, clusters of neurons together, which might come from very different parts of a model. And some features will overlap, right? So some neurons will participate in decisions on certain topics where other those same neurons might participate in other features.
A good way to think of that is if I ask you to read an article or if I [00:34:00] ask you to write an article, they're topically related to that same thing, but they'll invoke completely different sets of neurons in your brain for those two skills. Certainly if I ask you to read it aloud, right? Because now you're speaking, it's a different modality, but some of the neurons will be similar.
Some cognition that's happening similar to understand the words. whether you're reading it aloud or if you're reading it in your head. And so that's definitely one way to think of features is that they're groupings of these neurons. And so your analogy is fine, too, where the submodels you can think of in, in my example, because I had 135 of them on that ship, there's a lot of them.
And so you could say, hey, groupings of these submodels working together is kind of like a feature. So just think of it as a group or a cluster. It's a pretty simple concept when you think about it that way. And don't worry too much about the technical definition.
Mallory Mejias: Are there any mainstream AI models that do not use this mixture of experts architecture?
Amith Nagarajan: Yeah, most do not. So, other than GPT 4, I'm not personally aware of other models that are doing that. Gemini probably does, but I don't believe there's been a disclosure on that. But GPT 3.5 and prior GPT series were [00:35:00] single, single models. Essentially, Llama 2 is a single model. So MOE is not a prevalent thing yet, but I think it will be for all of the mainstream LLMs and, and over time, especially as you get to multimodality.
We've talked about that on this podcast and many other times inside cars, blogs and additional. Now we spoke about that a lot. Multimodality is where an AI model can both intake different types of content like text, video, audio and output different kinds of modalities. It can speak to you in audio. It can generate videos.
Of course, it can generate text. And so if you think about multi modality, it's a very natural thing to say, well, you might have many sub models. So that could be one approach. In fact, GPT 4 does in fact do that. When you think about how it has chat, GPT can talk to you, Chat GPT can You know, I'll obviously deal with you in text.
It can generate image uh, in a way you can kind of see that working where you know, for example, dolly is the image generation. That's not part of the GPT 4 MOE but I'm just using that as an example where [00:36:00] the end product to you as a consumer is an integrated experience, but you have all these different parts working together.
Now, what Google said recently with their Gemini release, and this is what they said initially when they announced Gemini in the spring is that they were training one big model initially, at least it seemed to be one big model, on multiple types of content, whereas GPT 4 was trained on text, DALLE 3 was trained on images and so forth, and then they're brought together.
Which approach is better? It's going to be interesting to see how that unfolds. I think that models that have an intuitive understanding through their training of multiple modalities could be very powerful. We'll see how that works.
Mallory Mejias: At the top of this segment, you talked about choice, and I think at a glance, choice can be a great thing, right? Having an abundance of options, being able to choose a model that best works for your association, for your organization, but I also think this idea of choice can be really daunting when it seems like every day we have another model popping up.
And so I'm wondering for leaders that are [00:37:00] listening now, assuming that they've kind of gotten past that first hurdle of learning and education and getting their staff on board. What would be your thought process to find a model that works for your organization? What are the steps that you would think through, Amith, to select 1 of these many models that's out there to experiment with?
Let's say.
Amith Nagarajan: I think for the vast, vast majority of associations, except for perhaps the largest ones of the others that may be pretty substantial, just happen to have a very technical team which is, I would say probably less than 10 percent of associations, probably less than 2 percent of associations are not going to go out and do that themselves right now.
Maybe in 2024, there will be situations where they'll start to do that directly. Mostly though, associations are gonna work with vendors who are building stuff for them or buying products from companies like Betty Bot and others. And that's great. But the thing that you need to do as an association leader is know about this stuff and ask good questions.
So if you're gonna work with a vendor to build an application for you, talk to them [00:38:00] about the models they're gonna use. Don't just say, oh, well, it's a technical decision. We'll let the vendor make it. That's not a good approach because you need to be, you need to be aware of what the engine is that's going into your car, right?
You need to understand what that thing is, and you need to understand how the solution is being architected to give you optionality over time meaning that let's say OpenAI is great right now and it's the right model for you, and you wire that up into your business What happens if OpenAI goes out of business, right?
They had something of a near death experience recently when all the staff were planning to leave when Sam Altman and Greg Botkin were, were out of there. Obviously they've recovered from that for now, but the point is, and by the way I'm very bullish on OpenAI to be clear, I'm just using them as an example since everybody knows about them, but let's say they were gone.
What happens to your business? Well, the answer can't be, we're dead. The answer has to be, we actually have a plug in replacement. Essentially, we know how to replace this if we need to. The really nice thing about this technology is unlike things like operating systems or databases, [00:39:00] while there is obviously some close coupling and the way you build solutions on top of these it is possible to build an architecture where you have plug ability and you can swap in different models.
So my goal in talking about this topic for the association audience, the vast majority of listeners is again, it's important to be aware of all the advancements because this can affect how you go about thinking about solutions you buy or you hire people to build. And if you are in that smaller group of people who are building on your own I would definitely, going back to Mallory's question, I would definitely experiment with Mistral.
I would experiment with Llama. You can host these things in Azure. You can host these things in AWS. You don't have to do the, do the work yourself to set it up. And there's plenty of other ways to deploy AI models like this, and there's literally hundreds of them out there. And a lot of people have taken these open-source models and they fine tune them further on particular, for particular use cases.
So, there's a lot of choice out there. Again, the main message I keep sharing with people is design with the mindset of change. Don't think about this [00:40:00] the way you think about buying an AMS and think you're going to keep it for 10 years. You're probably not. You know, you do well to keep the LLM you pick for two years, and that would be probably a really long time.
So you have to plan on change occurring, and that's an uncomfortable place for most associations who are used to the kind of set and forget mindset around technology.
Mallory Mejias: So it sounds like for most of our listeners, then learning about the models is the first step so that you can ask the vendors you're working with or hoping to work with the right questions.
Amith Nagarajan: Yes, you need to ask them, which models are using? Are they open-source? Are they closed-source? You know, what is your plan if that model is no longer available? Do you, do you have a contingency plan? You know, how would you go about changing it? What kind of impact would that have on us in terms of downtime?
Would that cost us anything? Those are some questions that I think you should be asking your vendor. And in fact, many vendors that are not necessarily just in the association space, but just in general, people are building these so called wrapper apps. And [00:41:00] these apps are really basic, simplistic wrappers around the open AI API.
So for example, you saw a proliferation of talk to your PDF type apps where it's like, oh, somebody said, I'm going to take your PDF. And I'm going to, you know, be able to analyze it with a chat GPT type conversation, and all of a sudden you saw 50 other applications or more like 500 other applications.
And then low and behold, of course, open AI unsurprisingly puts that right into chat GPT. And so when you deal with a lot of vendors that are simply wrapping open a eyes content or their, their API, they have no plan for that. They basically are just hitching themselves to OpenAI and that's all they have.
And they probably would be surprised by you asking that question, which makes it a really good question to ask. So of course it depends on the nature of the vendor and like what they're going to do for you. But you know, if you're hiring someone to build you like let's say an AI agent environment for like abstract submission, like we talked about in the past you know, that would definitely be a mission critical app that you want to really think through which model or which models you're going to be [00:42:00] using in that environment.
Mallory Mejias: Now moving on to topic three, we are going to talk about the EU and its new AI Act. The European Council of Presidency and the European Parliament struck a deal on the first rules for AI in the world, or at least that's what the headlines say, with its provisional agreement on the Artificial Intelligence Act.
This legislation, achieved After intense negotiations, aims to ensure that AI systems used in the EU are safe and uphold fundamental rights and EU values. It's a historic move that sets a precedent for AI governance worldwide. The AI Act introduces a risk-based approach to AI regulation, categorizing AI systems based on their potential to cause harm.
High risk AI systems will face stricter rules, while those posing limited risk will have lighter obligations. The act also allows the use of high-risk AI systems for law enforcement with appropriate safeguards in place. Additionally, the act proposes a new governance structure, including an AI office [00:43:00] within the European commission to oversee advanced AI models and enforce common rules across member states.
Other provisions that are worth noting- there's an obligation for deployers of high risk AI systems to conduct a fundamental rights impact assessment before using an AI system.
High impact foundation models must comply with specific transparency obligations before market placement. The AI Act sets fines for violations as a percentage of the offending company's global annual turnover or a predetermined amount, whichever is higher. And the agreement has provisions in support of innovation, including AI regulatory sandboxes for testing innovative AI systems in real world conditions.
Amith, what are your initial thoughts on the first rules for AI, perhaps in the world?
Amith Nagarajan: Well, I think it's really interesting and for the EU to lead the way makes sense. They tend to be very forward in terms of the way they think about technology regulation, certainly in the arena of privacy. That's where they've led the way globally for [00:44:00] for some time. I think it's good that the EU has done something and that they have at least some degree of clarity around the expectations because in the absence of this act there was a lot of speculation on what you'd have to do to be compliant.
And the EU has been, you know, pretty heavy handed in terms of fines they've levied on technology companies over time. You could argue that they haven't been heavy handed enough as well perhaps, but so you wouldn't want to operate in the EU unless there was some legislative framework that specified what you could and couldn't do.
And so some companies actually have just turned off their systems in Europe. They've said, no, you can't have access to A, B, and C in Europe because we just don't have to deal with it now. And some companies will continue to do that. But at least now they know what they're getting into. So if you're going to operate in Europe, if you're either gonna have people there or sell your services there, you at least have a general framework that can give you some guidance on what the expectations are and what you need to do to provide these services in compliance with the EU's legal system.
So that's good. So hopefully that will actually [00:45:00] open up the EU market more to foreign developers like companies from the States or wherever else. To be willing to consider doing business there. You know, if we were doing work in the EU, I'd be very concerned right now with any of our SAAS AI companies, simply because until now there hasn't been visibility into what the expectations are.
So, whereas in the United States, it's similarly murky, but at the same time, we don't have a history like the EU does in terms of the nature of regulation around technology, which once again, that's not a statement as to whether it's good or bad. You can argue both sides of it. But it's just, you know, what you'd expect in the US is a little bit more of a freewheeling environment. Whereas if you have any recent experience in Europe, you know that you're not gonna have as freewheeling of an environment. So that is a really important thing. I'm hopeful this will result in more investment in Europe in AI because I was concerned that as obviously one of the largest economic regions in the world and one that's extraordinarily culturally important and obviously close to us here in the United States in many ways they were being left behind [00:46:00] in terms of AI. So I think it's really good from that perspective. As far as the actual substance of the act, I think only time will really have the ability to tell us a whole lot. The idea of having categories of AI systems, I think makes general sense.
But of course, as usual, the devil is in the details. And the question is, how do you interpret those categorical definitions? They provided definitions of those categories. But of course, if you're high risk, but you want to argue that you're not Then you will probably find a way to try to define yourself as not high risk and so on.
And there might be reasons to call something, oh, we're really only testing. We have a sandbox for innovation and testing. We're not yet deployed. But our definition of that's a little bit different than your definition. So it's going to take time. It's going to take case law it's going to take, you know, some experience.
And of course, the challenge with all that is the speed at which AI is moving. AI is moving so, so fast. And legal systems, EU or anywhere else, do not move quickly, typically. So, uh, it's going to be super interesting to see what happens. I think that the [00:47:00] government plays a very important role in AI. I like seeing governments take some kind of action that's measured.
I don't want them to freak out and try to stop progress. I think this was a fairly balanced perspective overall from what I've read of it. I'm not an expert in the act, but from what I've read about it, it seems like a pretty good step. So hopefully Europe will be nimble with this and they will rapidly amend the act as they learn from it and hopefully they'll be leading the way in terms of what other
regions of the world and other countries like the United States might choose to do. My, my thought on associations and Europe is that you have to look at it from the viewpoint of where you operate. So if you're an American association or an Australian association or Canadian and you have customers or members in Europe, you have to pay attention to this stuff because it'll affect services you offer if you have any AI features.
Now, are you on the you know, the radar of the EU? Very, very unlikely. So you're probably not going to be someone that is caught in being out of compliance, but you still need to pay attention to it anyway. So, [00:48:00] if you operate in Europe and you know, some of our companies obviously do uh, you have to be very thoughtful about what this means.
Mallory Mejias: That's a great point. When I was researching this topic, I read, I believe that this was going to take, you know, a few years to kind of come into action and be enforced. And it's crazy to think about, because in a few years, I can only imagine how much this act will need to be changed to kind of keep up with the times, given how, we have to update this podcast basically every hour to make sure we're up to date with the time.
So, it will be interesting, I think, to see how the AI act shifts and plays out.
Amith Nagarajan: Definitely. And, you know, from my perspective I think again, you know, whether a lot of times the, in something as dynamic as AI, you have to have a lot of discourse. And my hope is that there will be a lot of debate. There will be a lot of dialogue on a variety of different concerns. And some of the concerns might be moving too slowly.
Whether that's moving the industry too slowly or moving the regulation too slowly. But the world is a very dynamic place. And Europe taking a leadership role in this is culturally [00:49:00] right for Europe. So I think it's, it's good from a European perspective to have something in place. They just have to have the mindset of adaptability and changing it over time.
And I think as an association, you know, whether you have the word American or Australian or Canadian in your name, or if you're a European association listening in you've got to pay attention to the global landscape of the rules and regulations. Hopefully, there will ultimately be some consensus globally around certain basic rules that are required.
Uh, and maybe it becomes a UN thing. I don't know what eventually happens with this. And, you know, countries sign on to those. those regulations, or those standards. But, you know, ultimately this is a topic that I think is super, super important for everyone to be aware of, particularly if you have operations active in the region.
Mallory Mejias: Last week, we talked about AI governance and particularly that containment just is not an option for AI. How do you see this act play out in that conversation? Do you think this is an attempt at containment or do you feel like you mentioned that it's balanced? So I'm assuming you feel like it's [00:50:00] pretty open in that sense.
Amith Nagarajan: I mean, it is in a sense in that they have provisions for these ideas of innovation and sandboxes, but they do have, you know, categories of AI systems that have to uh, you know, achieve certain levels of impact assessments and also for the higher risk categories, I think there's a number of things you have a number of hurdles you have to clear.
And so the question is, is that you know what's going to stop someone outside of the EU from moving a lot faster and the answer is nothing. Of course, you know, a company in China or the United States or anywhere else for that matter, can participate and move a lot faster. So it's not so much containment isn't something that you has control over, nor does the United States.
And frankly, even if all the global, you know, world leaders got together and tomorrow morning they passed an act that said something, that's still really not enforceable. So containment ultimately, I think, isn't a practical solution. But you know, you can say, okay, well, that, that might be true for a lot of technologies, right?
You could say, well, guns can't be contained and that's a problem. It's a problem in the United States. Perhaps more so than in Europe, right? But statistically, it is clearly [00:51:00] but you also have a question of enforcement of laws. So that's the side of it where Europe is known for taking you know, very, very strong approaches to enforcement around technology companies not complying with rules, whether it's antitrust or its privacy regulations.
So, you know, we'll see what happens. I think if people realize that if you get caught not following the rules that it's, you know, billions of dollars or puts your company out of business, or maybe there's even criminal penalties that could have an effect. So I think containment uh, is an open question.
I'm skeptical about whether or not containment is possible, as I've mentioned more than once before in this podcast, because I think there's always gonna be actors out there moving after going after a prize as big as AI that are going to skirt right, whatever regulations that there are out there, but doesn't mean that it's the wrong thing to do.
It's still, you know, there has to be leadership in terms of what a country or a group of countries thinks is the right way to approach anything in technology because this is ultimately a societal and a cultural question that has to be answered.
Mallory Mejias: [00:52:00] Well, it will be really interesting to see how AI plays out over the next few months, how it plays out in 2024. And if you want to make sure that you are keeping up with the latest advancements, you should definitely check out Sidecar's AI Learning Hub. Reminder, you get access to flexible on demand lessons, and we regularly update those.
Depending on the latest AI advancements, you'll get access. It's two weekly live office hours with AI experts, and you get access to a community of fellow AI enthusiasts. So definitely check out the Learning Hub at sidecarglobal. com/bootcamp. Amith, thank you so much.
Amith Nagarajan: Thank you very much.
Mallory Mejias: See you next week.
Amith Nagarajan: Thanks for tuning into Sidecar Sync this week. Looking to dive deeper? Download your free copy of our new book, Ascend, Unlocking the Power of AI for Associations at ascendbook.org. It's packed with insights to power your association's journey with AI. And remember, Sidecar is here with more resources for webinars to boot camps to help you stay ahead in the association world.
We'll [00:53:00] catch you in the next episode. Until then, keep learning, keep growing, and keep disrupting.

December 14, 2023