Show Notes
Join Amith and Mallory in Episode 6 as they explore the speculation around OpenAI’s Q*, not to be confused Amazon Q, a new AI chatbot assistant. They'll also dive into the idea of reimagining organizational structures through AI-integrated workflows.
Let us know what you think about the podcast. Drop your questions or comments in the Sidecar community: https://community.sidecarglobal.com/c/sidecar-sync/
Join the AI Learning Hub for Associations: https://sidecarglobal.com/bootcamp
Download Ascend: Unlocking the Power of AI for Associations: https://sidecarglobal.com/AI
Join the CEO AI Mastermind Group: https://sidecarglobal.com/association-ceo-mastermind-2024/
Thanks to this episode’s sponsors!
- AI Learning Hub for Associations: https://sidecarglobal.com/bootcamp
- rasa.io: https://rasa.io/
Articles/Topics Mentioned:
- OpenAI researchers warned board of AI breakthrough ahead of CEO ouster, sources say: https://www.reuters.com/technology/sam-altmans-ouster-openai-was-precipitated-by-letter-board-about-ai-breakthrough-2023-11-22/
- AWS Unveils AI Chatbot and Assistant, Taking Aim at OpenAI: https://www.theinformation.com/briefings/aws-unveils-ai-chatbot-and-assistant-taking-aim-at-openai
- Reshaping the tree: rebuilding organizations for AI: https://www.oneusefulthing.org/p/reshaping-the-tree-rebuilding-organizations
Social:
Follow Sidecar on LinkedIn: https://www.linkedin.com/company/sidecar-global
Amith: https://www.linkedin.com/in/amithnagarajan/
Mallory: https://www.linkedin.com/in/mallorymejias/
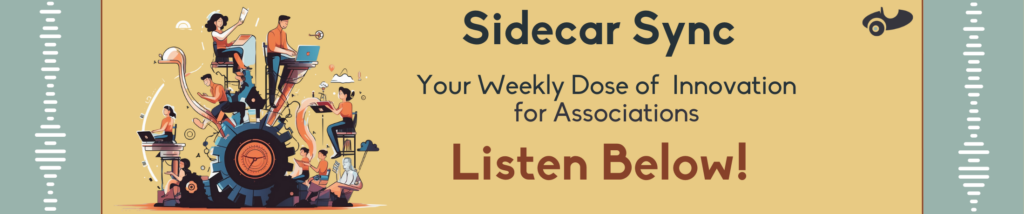
Amith: Greetings, everybody. [00:01:00] Welcome to the latest episode of the Sidecar Sync. As usual, we have a ton to talk about. My name is Amith Nagarajan. I'm your host. I'm here with Mallory Mejias, my cohost. Before we get into a whole bunch of exciting things that are happening in the world of AI and how they apply to associations, I wanted to take a minute to thank our two sponsors for today's episode.
The first one is Sidecar's very own AI Learning Hub. You can access information about this AI Learning Hub at sidecarglobal.com/bootcamp. The AI Learning Hub is a way for you and your team at your association to come up to speed on AI, and importantly, stay up to speed on AI. When you access the Hub, you get 12 months of access, and lessons are continually updated.
At the moment, there are over 30 lessons of content. And in addition to that, we have a discussion forum and access to a weekly office hours with AI experts so you can ask questions live. This is a great way to get the new year started and to get ahead of the new year. So join now. [00:02:00]
Our second sponsor today is rasa.io. Rasa.io is an AI powered email newsletter tool built specifically for associations. And what it does is it provides a truly personalized one to one email for every single one of your email recipients. Rather than sending the same newsletter to every single person, rasa.io learns each of your recipients and personalizes the content for every single one of them, resulting in dramatically higher engagement and higher satisfaction. Learn more at rasa.io.
Mallory: Thank you to our sponsors and I also want to add for that Sidecar AI boot camp, that we do have a special offer right now for the first 50 people who sign up, you can get lifetime access for the price of the annual subscription, which is $399.
So definitely check that out. We have a few spots left for that special offer. Amith, how are you doing today?
Amith: I am doing great. It's fun to be back at [00:03:00] it and to have a holiday week behind us. And you know, I don't think AI took a holiday though.
Mallory: Exactly. I don't think AI ever takes a holiday. Every week I'm wondering, hmm, I wonder what the news items will be.
I wonder if there will be enough news for us to talk about. And there's always plenty. That's what I've learned. So today our first topic is Q*. We want to talk about all the buzz speculating about a possible new model known as Q*. This model, potentially developed by OpenAI, has been a topic of intense discussion, especially in the context of Sam Altman's recent ouster as CEO of OpenAI.
Q* represents a potential leap in the pursuit of Artificial General Intelligence, or AGI, a type of AI that can autonomously perform a wide range of economically valuable tasks, surpassing human abilities. What makes Q* particularly intriguing is its ability to solve mathematical problems, a domain where AI has traditionally struggled.
Unlike existing AI models that excel in language translation or [00:04:00] writing by predicting the next word, Q* has shown promise in mathematical reasoning, a skill that requires a deeper level of understanding and logic. The possible development of Q* has not been without controversy.
It may have been one of the factors leading to Altman's temporary departure from OpenAI Apparently researchers at OpenAI raised concerns about the potential dangers of such a powerful AI fearing that without proper understanding and control it could pose a threat to humanity. I want to mention that this is all speculation at this point, and nothing has been published officially.
Amith, I have never personally run a math problem through ChatGPT. Maybe you have, maybe you haven't. I will say that initially hearing this, it doesn't seem that shocking, right? For me at least, that AI could do math problems. Can you explain what's so special about this?
Amith: Sure. Well, you know, interestingly with language models, one way to think about it is that you can get a really compelling response from a language [00:05:00] model, but it might be different every single time you prompt it.
Even with the exact same prompt in the same model with all the same settings, you could get a different response each time. And that's okay with a lot of things, certainly in conversation and writing essays and crafting all sorts of valuable outputs from language models. But in math, there's one right answer.
And so, in order to get to that right answer, you have to actually typically go through a stepwise, you know, process. There's a reasoning process. Language models are essentially predicting the next word. That's essentially what they do. And we talk about that a lot on this podcast. And others have probably, you know, shared similar background information.
But it's important to just quickly recap that the way a large language model works, is it has this, you know, incredible statistical data set on words, essentially on language. And when you put a prompt in, it's looking to complete the next word. There's obviously a lot of science behind making that actually useful, but it's called an auto aggressive token predictor.
[00:06:00] And so that type of model doesn't actually have any understanding of what it's creating. Sometimes it might appear like it's reasoning where, for example, it can emit a quite complex answer on something that looks like a step by step type of thought process. But really, what it's doing is just predicting language based on what it's seen in its training data.
In comparison what's not, this is not known, but what's, what's being speculated about Q* is that it uses a different approach, something that actually influenced the name called Q learning, which essentially is an AI approach that requires a model to be able to do step by step reasoning. So to solve many math problems, even grade school math problems, you have to think through a problem piece by piece or step by step and evaluate a lot of possible answers. So that's one part of it is this idea of What some people are referring to as the tree of thought, which is basically this ability to explore different branches of potential solutions and then continually iterate through those potential [00:07:00] branches until you either achieve a correct or incorrect answer.
So that's part of what's potentially new about this type of model. The other part of it is that there's this idea right now with language models where you just want to get the answer as quickly as possible. And in fact, we as consumers of these models, when it takes a little bit of time to get our response, we probably get annoyed because we're used to instantaneous responses to everything. But the question has been asked by the research community, what would happen if we gave these models more time to think? It's kind of like if you went to a person and said, hey, give me an instant reaction to 2 plus 2.
When you say the answer is 4, you're not actually computing 4, you just know that it's 4 intuitively, because that's essentially your intuitive, reactionary, pattern based type of response, which is actually very similar to how language models work. In comparison, though, if I gave you a more complex problem that you had to work through, you need to take the time to think through it, break down the problem into component parts, solve those problems and then generate the correct answer.[00:08:00]
You need a little bit of time to do that. And so what's been hypothesized in the research community is this idea called test time computation. Which is basically just giving models more time to think. And so, between giving models more time to think, which there is research published on, and this idea of tree of thought and Q learning you have the makings for what essentially becomes a broader capability to reason. Math problems are a great domain to explore because they do require reasoning and they're actually very simple to evaluate.
They're either right or wrong. What's been said through the speculation about open AI and Q* is that Q* apparently was able to solve math problems, but specifically math problems it had never seen before. Now, the reason that's significant is because, again, language models simply use what they've been trained on to predict the next word or predict the next token and in the context of mathematical reasoning to be able to solve problems you've never seen before, sure there are some patterns that might lead you to be able to guess, which is what [00:09:00] language models doing. But to get the correct answer every time on math problems you haven't seen is a different order of capability. And so that's what the excitement is about.
The only other thing I'll say just to open it up is that you can extrapolate from solving grade school math much, much greater capabilities because once you can reason in this fashion, you're thinking more along the lines of what's often referred to as system two thinking, which is this much more complex, logical process that we go through where there's much more, really, mental horsepower being thrown at it.
So that's the way I would tend to describe it again. I think that this type of work is being done by the research community very broadly speaking across all the major labs. They're all focused on this. Apparently OpenAI may have had a breakthrough in this area internally, and that's perhaps what led to some of the recent drama we've all been hearing about.
Mallory: What you're saying makes me think of an example I heard from Thomas Altman, who is a co-founder of Tasio and one of the creators of Betty Bot, in a [00:10:00] prompt engineering webinar he led, he gave an example of a really simple math problem, I don't remember exactly what it was, but something really simple, something like you have five apples, you give two away, how many do you have left?
And at first, Chat GPT could not solve it, but then when he prompted it to think step by step through the problem, that's the only way it could generate the correct answer. So I guess it does make sense that this model, potentially Q*, doing mathematical problems and getting the right answer every single time that is pretty impressive.
Amith: Yeah. And what you're referring to in terms of a prompt design asking the language model to think step by step actually does result in more compute more compute time being applied to the problem. It still doesn't provide the language model, the ability to truly reason in the sense of q learning, which is this idea of essentially exploring all the possible solutions to get to the correct one.
It is still doing essentially a probability distribution to just guess the next word. It's just a better guess because you've prompted it in that way. And so you essentially are starting to simulate reasoning [00:11:00] through current language models with better prompting, which is very powerful but it's still not entirely reliable.
And so the idea is, is that if we can get AI models to be essentially 100 percent good at math. Then there's all sorts of unlocks in the world of science and scientific research, particularly medical research. It can be very interesting to see what happens when these AI models at the scale they can compute are able to tackle problems like finding novel compounds that might be cures for diseases and things like that.
So, it potentially has a tremendous amount of power in terms of novel applications, as opposed to language models, which really know how to replicate what they've already seen. This type of reasoning is actually how scientific discovery works. And so that's, I think, where a lot of the excitement and the fear comes from because it's, it's a different order of capability than what we have today.
It's not just a faster, more powerful language model. It's really a different type of brain, essentially, for the AI.
Mallory: That makes sense. So I'm understanding why Q* is so impressive. I'm [00:12:00] still not fully understanding, though, why it would be seen as scary. I understand what you're saying, that this is just a different level of capability, but why would something like Q* be scary enough to have this whole debacle at OpenAI, to oust its CEO?
What, what are the concerns there?
Amith: Well, if, if that was indeed the case, where the Q* model and the advanced, you know, efforts to commercialize it, Were an element of the board's decision to let Altman go. You know, the thought process would be that the folks that are on the board of OpenAI or previously were on the board of opening, I should say we're essentially like pulling the safety hatch, you know, saying, Hey, hold on a sec.
What's happening here might actually be an existential threat. Let's pause. And the theory is that Altman and Brockman and others were moving as fast as possible to commercialize. So the idea would be potentially that this model, if it is truly novel and OpenAI is the only lab in the world that has something like it, which may or may not be true, right?
There's a lot of very smart people with a lot of money exploring this in [00:13:00] parallel across hundreds, if not thousands, of advanced research labs. But to the extent that OpenAI believes that it really is in that position, perhaps they didn't want to let the genie out of the bottle. They didn't really know exactly what it means.
So it's partly actually your own question, Mallory, that clearly this is a new type of capability. It's considerably more powerful than just, you know, token or word prediction. But what does that actually mean? And so the safety advocates might be saying, hold on a sec, let's test this, let's evaluate it, let's theorize on it, let's think about what this means before we commercialize it when you can't really put it back in the bottle.
That's entirely speculation on my part, but I think that could be a pretty reasonable debate between AI safety advocates and AI, you know, accelerationists.
Mallory: If Q* potentially became available to the public of course, to associations as well, what potential applications would you see for a model like that?
Amith: Well, I get excited about the application of this type of advanced reasoning model to problems in the association domain related to [00:14:00] interpreting the content the association has.
So right now, the state of the art would be to take the content the association has, so reams of historical journal articles, proceedings from conferences, blog posts, possibly content from an online community. You take all this rich proprietary content that an association has and you train essentially a language model around it.
And there's a variety of ways to do that. And that language model then is capable of interacting as an expert bot with your audience. So acting as an expert that can help your audience, your members anyone else who you give access to it to answer questions related to your domain. But it's, it's limited to the same capability as things like GPT 4, where it's essentially predicting based upon the input provided to the by the user.
So, for example, if I'm in a field where there's advanced research happening and we're exploring, you know, new approaches to solve problems, it will be able to help give you answers based on what's known, but it won't [00:15:00] necessarily be a good thought partner in brainstorming what's what might come.
And so this model or this type of model potentially could ingest all of the known examples of peer reviewed journals in a particular domain, which is essentially the accepted science for a particular domain. Learn that as example content. And then using the capabilities we've been discussing. Including this idea of Q learning or tree of thought, coupled with larger computational time windows to be able to reason, which could then lead to being essentially a thought partner in brainstorming what might come next.
So actually being part of inventing novel treatments in a pharmaceutical arena or coming up with novel materials that could be used to construct the next generation of batteries. Or in fields outside of science, perhaps in the accounting world, there might be applications where you really want the AI to be able to help you do computations relative to a [00:16:00] client you're working with.
So there's a lot of places where this capability I think could And could not only allow an association to do more powerful things itself, but to really supercharge that content. You know, we've been talking for a while through the book Ascend, as well as, you know, other outlets, how important it is for an association to rethink its business model and to think about its content and its brand and its community as assets that it can leverage in this age of AI. And certainly AI assistants that are experts in your content that can do like what GPT 4 does to are awesome. But when you add this to the mix, it goes beyond being an assistant and it really becomes like a lab partner that can, you know, work with you at that PhD level. So it's a different game entirely at that stage.
And I find that very exciting. I also really see the point from the AI safety advocates about really not understanding exactly what this type of capability might do. And so I get those concerns. What I would say about that is you know, I, I don't [00:17:00] believe personally that OpenAI is alone. There are a lot of other labs that are pursuing similar things.
We know from quotes from Sundar Pichai of Google that the Gemini model that's being developed, we don't have a timeline for it, has a number of capabilities that actually are very similar in concept to this. And that's just one company. You know, we know Amazon has a lot going on. Microsoft's developing its own models independent of OpenAI. IBM is doing tons of work. Really good work around AI. They have deep, deep roots in artificial intelligence. And that's just a handful of companies. There are literally hundreds and hundreds of labs out there exploring this. So the reason I share that again and again is that first of all, you know, that's going to drive the rate of progress, whether we like it or not.
And so no individual organization like OpenAI, and frankly, I don't believe any individual government or even governments collectively are going to be able to stop that by saying, hey, let's pause on it. This stuff is going to come out. And so I think that, you know, we have to be conscious about that.
We have to be thoughtful about it. I do think it's worth [00:18:00] absolutely thinking through this, but at the same time, it's not up to any particular organization to decide whether this thing is going to come to, to light, because it might be three months later that someone else brings it to the market, but it's going to be coming.
And these are things that the fundamental research that's going to drive this stuff is out there.
Mallory: You just mentioned Amazon, so I think now is a good time to say that Q* should not be confused with Amazon's new AI assistant. Just this week, actually, Amazon Web Services unveiled its own AI chatbot known as Q, which is said to be a rival to ChatGPT.
It appears to have a similar pricing structure to ChatGPT plus at 20 per month per user. It will be able to access proprietary business data from applications like Microsoft 365 and Google Drive. It's not clear exactly which AI model is powering Amazon Q, but the FAQ section indicates it's powered by, quote, various foundation models from Amazon Bedrock within Amazon Q. Amith, will you be trying out Amazon Q as soon as it's [00:19:00] available?
Amith: I'm sure I will be. I mean, I, I try all these things as they come out just because it's part of what I do right in order to understand what people are doing. I wouldn't necessarily recommend everyone try to jump on it and try it out immediately out of curiosity.
I think it'll, it'll be seen very quickly. Are there some novel capabilities that exist in Amazon queue that are not available in Chat GPT in other places? In some senses, this is highly predictable, right? Because a major technology company doesn't want to not have an offering in this major area.
This has become a major software category. You know, OpenAI on its own is at over 1.5 billion annual run rate on on their product. And, you know, it's primarily the Chat GPT subscriptions, and I think the API portion is a small minority of that from what I've heard. And that's growing like a weed, right?
So there's a massive opportunity out there. Microsoft's obviously all over this with Copilot, Google's doing the same thing with Duet. Amazon, up until now, has had some infrastructure available for software developers through Bedrock and through other more traditional [00:20:00] AI services they've offered for a long time.
But they haven't had a consumer facing chat tool. One thing I'm curious about is how does this tie to Amazon's Alexa service, their voice assistant that has been ubiquitous in a variety of ways over the last several years. I would imagine that they're thinking about how to tie it together if it's not already, you know, designed that way.
Because the capabilities of the chat assistants like ChatGPT far surpass what Siri and Alexa and Google Assistant have historically been able to do. And so it's only a matter of probably months before you see broad consumer adoption of these more powerful models, you know, through Siri, through Alexa.
So we'll have to see what happens there. I think one thing that's really important, though, to point out is that Amazon, when they, they are developing their own models. So Amazon, like all the other major technology players, is developing its own models. However, they've also taken an approach of essentially saying, we're model agnostic.
We're gonna work. With a bunch of these other companies to make their models [00:21:00] available on the Amazon Web Services platform, specifically this thing called Amazon Bedrock, Microsoft has taken a similar approach with Azure, even though they're deeply partnered with OpenAI, they have their own models.
They're offering models from third parties. And I think that's really smart. I think that's an important thing for associations to pay attention to because associations have been somewhat shocked, I think, by all the OpenAI upheaval. And part of what I've been saying to people is, first of all, I don't think this is gonna be unusual in the craziness of AI because there's so much at stake, there's going to be more drama like this from different companies. So in that sense, it's good to have some sensitivity to it, but it's also good to have been, you know, given this episode to further tune us into it.
And why I point that out is you don't want to hitch yourself to just one vendor. So, I know people who are building specifically on OpenAI. And that's great in the sense that OpenAI has, you know, really the most advanced available models via API today. But you should be thinking about how to insulate yourself from change. Just [00:22:00] like, you know, you don't want to be wed to any particular CRM vendor too tightly, although associations painfully find themselves exactly in that place.
Associations now need to think about this new critical piece of software infrastructure and how to create an installation layer between themselves and particular vendors. And so Amazon Bedrock is one way to do that. Azure AI services is another way to do it. There are a number of techniques you can use from a software perspective, but I think it's smart of Amazon to have this blended model approach.
And we're seeing it appear here in their latest consumer product.
Mallory: I can't imagine there's enough room in the AI space to have a Q and a Q*, but I guess that is uh, to be determined.
Amith: You know, it's funny because who knows Amazon is known for being quite nimble. And so perhaps, you know, this is like one of the most one of the best examples of newsjacking where they're like, hey, people are talking about Q*. Let's call our thing Q. I doubt that I'm the same. That's somewhat jokingly. But uh, yeah, Amazon Q has nothing to do with what we've been talking about with Q *as Mallory already said. But it just appears to be an interesting new chat bot [00:23:00] opportunity that's out there. So it's, it's worth checking out when it becomes available.
Mallory: The next topic we want to dive into today is organization structure and artificial intelligence. So the concept of rebuilding organizations for AI integration revolves around adapting traditional organizational structures. Historically, organizations have been structured in a rigid hierarchical manner, making them resistant to rapid technological changes.
However, with the advent of AI, particularly advanced models like GPT 4, like we've chatted about, there's a growing need to rethink these structures, AI's ability to perform tasks that were traditionally human driven, such as analyzing data, generating reports, and even assisting in decision making presents an opportunity for organizations to become more efficient and innovative.
This shift requires a move away from these fixed hierarchies to more fluid and adaptable structures where AI tools are integrated into various aspects of the workflow. This topic today was inspired by an article that Amith and I read from Ethan Mollick called [00:24:00] Reshaping the Tree, Rebuilding Organizations for AI, and we will definitely be linking that in the show notes.
I want to give you all a brief overview of the example that Ethan gave in the article because I feel like it really sets the tone for this conversation. He said that he and his team, when they're designing a new feature for their core teaching platform, specifically in this case, making changes to a screen that gives feedback on game progress, they have this long, drawn out process to do that.
It typically takes one to two weeks. They have to gather information, get feedback, get consensus, and then do testing, and then get approval on any changes after that testing. You can imagine, Amith, hearing this, that sounds like a whole lot of meetings. And a whole lot of work. So one to two weeks sounds about right.
Ethan proposes using AI in this situation as intelligence instead of simply a tool. And that's what I want to get into now and explain the example that he gives. So, in the first step of that new process, using AI as intelligence, he gives ChatGPT, for example, a screenshot of the [00:25:00] platform that they use and asks ChatGPT itself to give that first round of feedback.
Then, sharing that feedback with your team, you can have everyone record their comments, concerns, and their own feedback using an AI voice transcription service. Then, you could put all these voice transcriptions of their feedback into GPT 4 and have it compile it into a nice meet table that you could hand off to the project lead.
From there, you could even have AI generate HTML prototypes of the proposed changes. And when you do finally meet, you could use that meeting as a real time building exercise to make changes using AI tools to these HTML prototypes so instead of just talking about the project, you can actually do the project while you're meeting.
He proposes that this whole new process might take 1-2 days as compared to the 1-2 weeks of the initial process. Amith, I'm curious. This was a really interesting article. Do you have any initial thoughts there? What do you think about this idea of traditional rigid structures [00:26:00] and changing them to embrace more AI driven workflows?
Amith: Well, I think Ethan Mollack's someone that we follow pretty closely. He tends to put out some really good content at the intersection of business. He's a professor of business at the Wharton School. He also happens to be, you know, super forward looking in terms of AI. He's been deep into the generative AI space ever since it really kind of burst onto the scene. And so he tends to have some really good insights. I think there's a couple of things that I'd like to point out about the article. First one quick comment is this idea of multimodality. So Ethan's describing the use of screen interaction, or at least screenshots from a software application and feeding those to an AI for a feedback loop.
And that's something that a lot of people don't necessarily realize is available even today. In Chat GPT, you can upload an image. And ask Chat GPT to do stuff based on that image. You can tell it simply to describe the image, which is an easy example. But you can also say, hey, here's an image, evaluate the software, tell me what's wrong with it, tell me what could be made [00:27:00] better and so forth.
So very similar to what he's describing there. The generality of what the tools can do would support, you know, this kind of workflow. So multi-modality is one piece of it that's worth noting. And then the ability for the tools to actually generate software is also something worth pointing out that they're not only able to produce text output in the form that humans can read, but these models can create very compelling software capabilities that are essentially turnkey.
The last thing I wanted to point out is that we've spent some time on this podcast and other venues talking about agents and specifically multi-agent frameworks like autogen and what Ethan describes in his article is a perfect use case for a tool like autogen, which allows you to define multiple different AI agents, which are each capable of doing specific things and to orchestrate how they work together through conversation. And we actually have a new lesson on the AI Bootcamp that Mallory and I both mentioned that specifically explains how auto [00:28:00] gen and multi agent frameworks works. I'd encourage everyone to check that out. But the point about it is that the software tooling for this stuff is there.
That's really what I'm trying to point out. He's not talking about a future where software gets a lot better than it is now. In order to achieve these things, he's talking about stuff we have access to today. So the first thing I want to point out is actually how he closes out the article. He talks about the fact that today is, you know, things are moving really quickly and you can either move too slowly or too quickly when it comes to anticipating exponential change.
So coming back to your question of what associations can take away and how they might apply this to their organizational structure. The first thing we have to do in associations is we have to empower our team to experiment.
Too many associations are extremely rigid, have a command and control structure that is designed to mitigate risk. These structures are based upon the assumption of a very mature business model, a business model that [00:29:00] really is pretty, you know, deeply ingrained and doesn't need to have a lot of change.
So when you have something that you've done for a dozen years or for 50 years and you don't want change and you want to make sure they execute it exactly the same way every time that having a very rigid and hierarchical structure can make sense. You know, at the same time, what's happening now is so dramatic that it's really important to empower your team to be willing to experiment, to take experiments and to reward them for that.
So if I were an association CEO today, I would do two things related to this article. The first is, is I would make sure that my team has access to great education on AI. And I'd kind of push them hard to do that. I'd say, look, this isn't optional. Every single one of you has to do X number of hours of AI education in the next 30 days, like not the next year, I would push people to learn it now. And then I would give them both budget and freedom to experiment with it. Then I'd pull back the feedback from that type of [00:30:00] process and then learn about where opportunities like Ethan's example might exist in my own organization, where I can take a process that might require dozens of meetings and weeks of time and bring that down to days.
They are all over the place. There are tons of processes in the association world. that are exactly like Ethan's example where you might get 80 percent or even higher efficiencies, which is really profound.
So I think associations have to start though by breaking the rigid culture because the culture of essentially being intolerant of mistakes, which I see in most associations, and this idea of having to ask for approval for anything that you want to do a little bit differently will, it's, it's basically like a form of an immune system that's, you know, treating innovation as you know, an outside bacteria or something that needs to kill off.
And we have to change that in our culture if we have any chance of adapting to this type of AI innovation.
Mallory: I would say that I'm very fortunate to have had a good bit of AI education through my work with Sidecar, through my [00:31:00] work with the Blue Cypress family of companies, and I still find myself getting stuck in this tool mindset in terms of using Chat GPT for one off tasks and not really thinking of AI as intelligence, like Ethan says. How do you recommend getting out of that mindset, and especially with associations, not only encouraging your staff to experiment, but also encouraging them to experiment in a way in a deeper use case of AI.
Amith: You know, Mallory, I think that this mindset shift going from thinking of this is a set of tools to really looking at it as intelligence is Ethan proposes requires probably a couple step process.
The first thing is you've got to get going. You can't think of it as this higher order intelligence and really have deeper innovation to completely reframe your business model and rethink entire processes until you've done little bits and pieces. So again, I'm probably a broken record, but you've got to start with some fundamental education and you've got to start with empowering your team to experiment.
But from there, there's some things you can do in your organization that I [00:32:00] think would shift the mindset to thinking of these tools as actually having a broader scope to act as intelligence. One of which is once you've had a little bit of experience, and this is key, having a little bit of experience of the tools so people have some level of understanding of what their capabilities are. Do a hackathon. A hackathon is something that in the software industry is pretty common. You get a group of people together, you go somewhere outside of their normal office or work from home environment. Maybe take a long weekend or a few days, you know, the middle of week, whenever, and you, you focus on a problem and you get people to really think differently about the issue.
You're not trying to solve your day to day problem. You turn off email, you turn off your phone, you turn off everything. And you think creatively in that environment, you'd be amazed at how many big ideas can come out of a hackathon type environment. Yes, it's certainly a great thing for software developers, but it's true for literally anyone.
We all get stuck thinking about the next task that we have to do in a way, we're kind of like a [00:33:00] language model. We're trying to predict the next task, right? Predict the next token that we get stuck in that rut. If we want to switch to the bigger picture type of world view, we have to give ourselves a little bit more breathing room, and that can be done through a hackathon.
That's a fairly big investment of time and energy and in some cases dollars. You could also do that just by dedicating an hour a day or even an hour a week to start. To think more creatively, work on something outside of your normal pattern. Read a book on a topic that's totally unrelated to this stuff.
Read a history book, for example and just think more creatively by forcing yourself to have different stimuli and then coming back to this idea of then how do you think of AI tooling as a higher order type of intelligence and where you use it. I think that you will find these bigger ideas coming to you pretty naturally if you expose yourself to different stimuli, if you give yourself oxygen in the room to think a little bit bigger picture, you're not just focused on the next production output. And of course, you've been at least somewhat educated on these tools so that you have an [00:34:00] idea of what their capabilities are.
Mallory: If you like the idea of a hackathon, but you want to make sure that you can get everyone on your team involved. You could also do an ideathon, which is something that we did earlier this year in May, I think, where we brought together association professionals to compete on teams to solve meaningful challenges that associations currently face using AI so they didn't actually have to build the product but they just had to really present the idea. They had some short videos and you know made logos and names for the product and you would be surprised what great solutions that they came up with and there was no programming required.
Amith: Yeah, and I think it's, it's a great point that the ideas themselves are incredibly valuable and, and that, that spurs a lot more thinking that can happen later on and the other thing, too, is that software development itself. Many of these things that we come up with this idea is obviously ultimately boiled down to how do you use software in different ways?
And a key point to make is that these AI models already are capable of producing pretty capable [00:35:00] software there. They're quite good at producing software. What's going to happen in the next 6 to 12 months is the next step of that, which is for someone who's completely non-technical to go to an AI and say, hey, I want you to build me a workflow that does these 20 things and to interact with it. Just like when you create a Custom GPT, which we've covered in the past. This idea of interacting with the AI to create another AI is very similar to what you're gonna do when you want to build software is you'll have a conversation quickly with an AI that's capable of going through the entire process. There may be humans in the loop to review the outputs and provide some feedback. But ultimately, the AI will be the manager of the process. And that's where that's where everything is heading with software development. So I think that associations who have considered software and technology to be a weak spot for them.
It doesn't have to be that way. Going forward, you have to free your mind up to think about these tools that are available to you. And these tools are just as available to small associations. And associations in general, as they are for [00:36:00] very large companies, this is one of the most powerful things about competition and the rate of progress is that it's not just that this stuff is getting more powerful.
It's that the cost is being cut in half or more every six months. So it's a really important point to think about, because many associations I speak with often will say, at least they've said historically, I love these ideas, but we don't have the budget. We don't have the energy to go after it. And first thing I'd say is, well, tough luck because the rest of the world might somewhat appreciate your challenges. Probably not at all, actually, but really they ultimately only care about what you do for them.
And so you have to figure this out. The other part, though, is that I don't think you're gonna figure it out until you get started with some basics. So, you know, to me, it's ultimately an idea that, you know, you go out there and you get started the basics and then know that these tools are available for you and know that you can, in fact, use these advanced technologies on even build software in the near future without the resources you've traditionally thought you'd have to [00:37:00] have.
Mallory: I think the key here when wanting to run an experiment similar to Ethan's to see if you can save you and your team sometime is thinking outside of the box. And so I think it's helpful to kind of think through an example of how associations might do this. I know for whatever reason, we typically always talk about on this podcast, a call for proposals and looking for speakers as an example of ways that associations could infuse more AI into that workflow.
I'm wondering if an association wanted to do that right now, maybe that first step would be after you get all the submissions, having AI do that first round of feedback on those. Do you think that's a good idea, Amith?
Amith: For sure. I think that's a fantastic idea. And there's a couple of things that happen with that is that first of all, by AI reviewing the submissions, you can have more consistency in the way that review occurs.
Typically committees that review large volume of, of abstract submissions for journals or for conferences, you know, they basically parcel out the work to lots of different people. And so how each of us may evaluate a [00:38:00] paper that's been submitted might be slightly different. There's certainly guidelines that are provided by the association.
Typically, in terms of how to evaluate the submissions. But you know, the computer is pretty good at following guidelines pretty consistently. Whereas we, as people aren't necessarily the best at that. And while AI systems reflect the biases of their training data, aka us. They also can be taught to identify those potential biases.
Whereas when human reviewers review content from people, there's a tremendous amount of, you know, biases that are built into the way that review process works. There's some immediate gains to be had from that, not just efficiencies. I would imagine that would save at least a couple hours in meeting time and then you could even go a step further and have the committee members record their own feedback with the AI voice transcription like Ethan proposes.
I think there's some big use cases there, but it's helpful to think about. You know, tangible examples of where to start.
Amith: Well, a lot of what happens in, you know, committee decision making is that, first of all, there is definitely a lot of group think going on. If you have a hundred slots at your [00:39:00] conference for sessions, and you have, let's say, 200 good papers out of 400 that were submitted, you've been able to easily get rid of 200 of them, but you're down to 200 really high quality papers that are being considered for these hundred session slots.
How do you pick the 100? And, and then what ends up happening is the group think in a committee tends to result in less risk being taken because the committee generally wants to achieve unanimous consent. Not always, right? And that's not the way the committee bylaws typically work. They might just require a simple majority, but still that group think is pervasive in the way people go about evaluating.
So they tend to do what? They go with the patterns they're accustomed to knowing are more likely to be successful because they don't want to be the committee that puts someone on stage at the conference who presented something that people look at and say, this is crazy. This is garbage, whatever. So there's that piece of it.
The other part of it is, is that people tend to accept content from people that they know or people that look like them or people that have similar names as those usual biases that all of us have, whether you know, we fight them really hard if we're aware of these things, but at the same time they're there.[00:40:00]
And so I think AI can be, people worry about AI biases, and I agree that that's something to be very thoughtful about. But I think as a collective group of people were more likely to have biases than the AI. And certainly the AI will be able to identify them more rapidly. So I think there's some interesting opportunities that will result in a better outcome, which is to me as exciting as the efficiency, if not more so and, you know, you'll also be able to process more ideas because right now, you know, you can't process an unlimited number of ideas coming in for a scientific journal or for an annual conference.
You have to limit that in some way because people are reviewing these things. So, potentially, we'll be able to find better ideas for a more diverse set of sources and produce more progress in these fields. So that, to me, is the most exciting aspect and one of the reasons I like talking about that example so much.
Building on that, but taking a slightly different path with a common process that associations have is providing customer service to their members and being able to do that with a high degree of quality.
Fast response times on doing it in the modality [00:41:00] that those individuals prefer, whether that be phone over email through the website. And this is an opportunity for really again, taking the same type of mindset that Ethan described in his recent article and looking at something that might take, you know, hours to take minutes or actually take zero time.
If an AI is in the loop in resolving a lot of common requirements or common requests. I frequently attend meetings with association CEOs and other senior leadership to talk about their AI Roadmaps. Um, I'm pulled in to have conversations about, you know, where are we going? Where's the low hanging fruit?
Where are the bigger opportunities? And a lot of times, you know, you see patterns in these meetings, regardless of the industry, the association serves. And providing high quality customer service is a big common element, which makes sense. Every organization has to provide good customer service. And so you oftentimes have some fairly knowledgeable people that are answering phone calls and, you know, answering emails because they know the association really well.
Sometimes it's like basic customer service stuff, like how do I get a [00:42:00] refund or how do I register for the event? But a lot of times it's actually more involved than that. It's people who are asking for really guidance on things related to the content or looking to connect with people or looking for the right events to attend or the right path in the education all the education offerings to pursue.
And so that's where I think I can be tremendous in reducing the load on the people, which in turn goes back to the earlier discussion we had about how do you think bigger? Well, if all you do all day long in your role is just process the next email process, the next phone call, you really don't stand a chance of thinking about other things.
You're just gonna be that next token predictor in human form. So what you need to do is give people a little bit more oxygen, a little more free time, a little more breathing room. And I think I can be used to automate a lot of the lower level processes. Thank you. And perhaps some of the people that are in that role in that lower level role in the organization might actually have some of the best ideas because they're the ones talking to your members all the time.
So I think there's some really big opportunities here associations can jump on.
Mallory: Do you think it's important to [00:43:00] measure outcomes with the integrated workflow as opposed to like the regular human only workflow to see if one's better than the other? Or are you pretty convinced that eventually having AI involved in the workflow will be better, ultimately?
Amith: Well, I mean, given that I'm an AI optimist and AI technologist, I definitely err towards the side of thinking it'll be higher quality over time. Not necessarily in all domains immediately, but over time. But I definitely am a fan of measuring everything, so... I would suggest as you work to automate these things from the initial prototype all the way through full deployment, you do measure the outputs, both in terms of the quantitative metrics, like speed to resolve an issue or certainly the qualitative aspects of customer feedback in terms of, you know, their satisfaction level.
You should also audit the work, even if you have something on full autopilot. You should audit the work that the AI does from time to time to basically keep benchmarking the quality that it's producing. That's super important. I don't think you would let a human team kind of do its own [00:44:00] thing without any supervision or any occasional review.
And I think the same thing applies to AI. So I think it's, it's really important to have as much objectivity as you can in evaluating both the performance and also the quality.
Mallory: Absolutely. Well, today we dove into the AI Qs. We had Q* potentially from open AI. We had Amazon Q. And we also talked about new organization structures with AI being infused. Amith, I want to thank you for your time today. And I also want to let all you listeners know or remind you that we do have the AI Learning Hub enrolling now. If you are looking for a self-paced flexible opportunity to keep up with the latest AI advancements, if you want to have fresh content on demand, and if you want access to a community of fellow AI enthusiasts, I would highly encourage you to enroll in that AI Learning Hub soon.
Reminder, we have that special discount for the first 50 to get lifetime access for the same price as an annual subscription. Thank you for your time, Amith. Thanks, Mallory. See you next week.[00:45:00]
Thanks for tuning into Sidecar Sync this week. Looking to dive deeper? Download your free copy of our new book, Ascend, Unlocking the Power of AI for Associations at ascendbook.org. It's packed with insights to power your association's journey with AI. And remember, Sidecar is here with more resources for webinars to boot camps to help you stay ahead in the association world.
We'll catch you in the next episode. Until then, keep learning, keep growing, and keep disrupting.

November 30, 2023